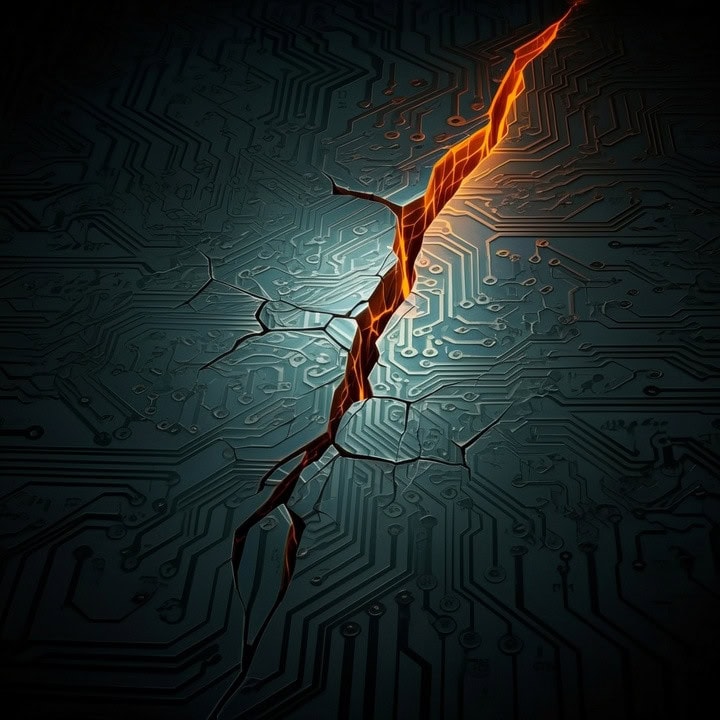
Establishing AI risk thresholds:
A comparative analysis across high-risk sectors
Artificial intelligence is a young field, and so is AI risk management. Instead of reinventing the wheel, we can draw some essential lessons from how risk is managed in other safety-critical technologies.
Much valuable research has focused on identifying specific risk thresholds for frontier AI or developing new capability evaluation techniques. To complement this, we zoom out and examine the building blocks of risk management frameworks that have made safety-critical technologies like nuclear energy and aviation so safe.
We first identify shared features of risk management in four other safety-critical industries (nuclear energy, food, pharmaceutical and aviation industries). We then examine the current state of risk management in the AI field and identify commonalities and differences with practices in other industries.
We find that in other safety-critical industries, government agencies commonly define acceptable levels of risk, weighing the advantages of adopting a high-risk technology against the risk of harm it entails.
Developers and providers must demonstrate their products are suitably safe, usually by testing for predefined conditions, then rating the consequential risk level and taking often specific action to mitigate the risk if necessary. Initial and repeated inspections by government and third-party oversight bodies ensure compliance.
In contrast, AI risk management relies mainly on private sector self-governance, with government oversight mostly based on voluntary commitments from developers. Under this system, risk management is based on observing a loosely defined state or condition possibly followed by a loosely defined risk mitigation action, without mandated and continuous external oversight to ensure adherence.