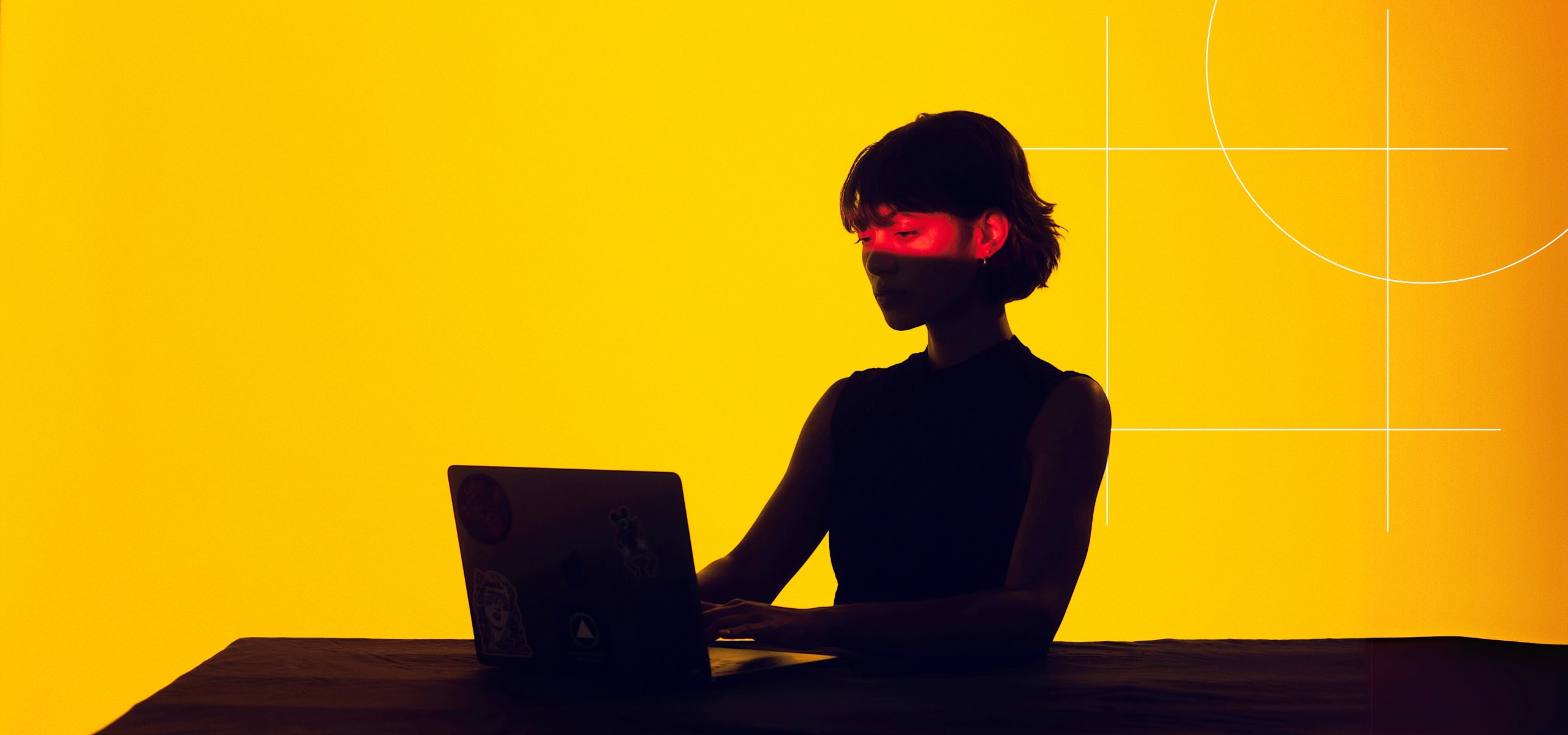
Beyond the AI hype
A critical assessment of AI’s transformative potential
Executive summary
Artificial Intelligence (AI) has become one of the most discussed and debated technologies of our time, with claims about its impact ranging from revolutionary transformation to overhyped disappointment. This report provides a structured, evidence-based examination of AI’s current state, its potential for impact, and the key uncertainties that shape its future. Rather than taking a position at either extreme, we break down the facts behind the hype, offering a clear view of what’s real, what’s exaggerated, and what remains uncertain.
Key takeaway
The evidence reveals a complex reality where genuine technological breakthroughs and speculative hype coexist. While the industry shows bubble-like characteristics—inflated valuations and widespread “AI washing”—it differs crucially from previous tech bubbles through a combination of clearly demonstrated capabilities (from protein folding to mathematical reasoning) and substantial revenue generation by leading companies.
The rapid advancement in AI capabilities – namely, the technical breakthroughs that have spurred the rush of attention and investment in this technology – appears sustainable, with new approaches overcoming apparent scaling limitations, though reliability remains a key challenge. Most notably, the convergence of accelerating technical progress, sustained investment from traditionally conservative companies, and dramatically shortened expert timelines suggests transformative impacts could emerge within years rather than decades—particularly through increasingly autonomous AI agents and automated research and development (R&D) processes. This compressed timeline makes our current policy and governance decisions disproportionately important for shaping AI’s trajectory.
How this report is structured
We explore AI’s trajectory by investigating the empirical data behind this sector, answering the following set of questions to form a comprehensive overview of where AI stands today and where it might be heading:
- AI’s global surge—How much attention and resources is AI receiving?
Examines the surge in AI-related media, policymaking, and funding, and whether current enthusiasm is warranted. - AI today—What is the current state of AI capabilities and societal impact?
Analyses AI’s capabilities, adoption, and measurable impacts, distinguishing where real progress is occurring. - Future AI trajectories—How soon could AI become transformative?
Investigates expert predictions, emerging technological developments, and key constraints that could shape AI’s future.
This report serves as a detailed companion to a more streamlined parallel FAQ document[1] for common questions and short answers. Within this longer report, we aim to provide in-depth analysis, additional evidence, and citations for those who want to dive deeper into AI’s trajectory. It is designed for policymakers, business leaders, researchers, and anyone looking to critically assess the evolving AI landscape.
Methodology
Before analysing ‘AI hype’, we first sought to understand how people currently talk about it. We reviewed literature discussing ‘AI hype’ and conducted informal interviews with colleagues and domain experts in the EU. The goal of these conversations was to ensure that our work aligns with the questions and uncertainties people in the EU actually have. In both literature and conversations, we found that the term lacks a commonly shared meaning.
In the conversations, some talked about the exaggeration of benefits on various levels—from certain AI start-ups’ valuations outpacing their profit margins, over AI infrastructure investments outpacing revenues, to deeper concerns around the advancements of the underlying paradigm or AI overall. Others focused on the aim to superficially ride the wave of enthusiasm (e.g., companies and philanthropic organisations using the AI label even when AI is not central to what they do). And again, others focused on the timeline of unlocking AI capabilities, or on what these capabilities might imply in terms of risks and benefits.
We found a similarly wide range of associations with the term ‘AI hype’ in literature: some define it as a state of intense enthusiasm and attention directed at AI[2], another scholarly definition characterises AI hype as any claim or narrative about AI’s performance that lacks rigorous empirical or theoretical support[3], and some scholars stress that hype is not merely a marketing buzzword but a social phenomenon: a collective state of anticipation. For instance, Powers (2012) defines hype as “a state of anticipation generated through the circulation of promotion, resulting in a crisis of value.”[4]
Given that we want to focus on what matters most for EU policymakers—especially for decisions that could shape the future for current and future generations—we concluded that it made the most sense to focus our analysis on the societal implications of current and future AI technologies, rather than narrower questions like whether certain start-ups are currently overvalued.
Policy-makers are tasked with protecting the value for current and future generations. When some experts claim that AI could bring transformative societal changes, that claim needs to be rigorously investigated.
The transformative impact from AI has varied definitions in the literature, ranging from describing smaller reorganisation of industries and effects that the AI already has[5] in our societies to a “transition comparable to (or more significant than) the agricultural or industrial revolution”[6]. Some authors are trying to hit the middle ground by setting the definition as “radical changes in welfare, wealth or power”[7], and others categorised levels of transformativeness ranging from influencing one economic sector, all sectors, or even redefining metrics of human progress[8]. Finally, some authors[9] tried to specify the criteria a bit more, suggesting that transformative impact might come either from economic transformation (where AI would account for the majority of full-time jobs worldwide, and/or over 50% of total world wages; in the similar line, Microsoft recently defined one of the thresholds as generating $100 billion in profit[10]); scientific/technological acceleration (where AI systems would be able to drive major scientific discoveries or engineer new technologies independently, such that those advances become the principal driver of a societal transition comparable to an industrial revolution); or AI-centric transformation (where AI irreversibly changes power structures or security worldwide, e.g. via ubiquitous surveillance or autonomous weapons). Given the relative lack of clarity, we’re using a broader sense of transformative impacts in this report, which is in line with the definition of “radical changes in welfare, wealth or power”[11].
To explore this question in a structured way, we broke it down into these sub-questions:
- AI’s global surge—How much attention and resources is AI receiving?
- AI today—What is the current state of AI?
- Future AI trajectories—How soon could AI become transformative?
This breakdown is based on the following logic: to have a societal justification for the resources and attention AI is receiving, the technology would either need to drive substantial changes already today, or there must be a plausible case for transformative societal changes in the near future. To answer those questions, the report takes a broad scope and connects different pieces of information rather than advancing the depth of any particular sub-question. We have, therefore, leveraged existing information and reports to present the strongest available evidence on each topic.
This report is not supposed to be a general guide for how to distinguish overhyped technologies from truly transformative ones, but rather a snapshot of the state of AI in relation to its potential for creating transformative impact. This focus distinguishes it from other popular reports mapping the state of AI (such as the Stanford AI Index[12]), while its depth and breadth distinguish it from many quick impressions and surface-level observations published in op-eds, blogs or on social media.
An alternative way of approaching the question of the degree to which AI has transformative potential is to look at a common set of specific claims about AI and assess these claims against evidence. This is what we have done in the our parallel FAQ document[13].
A note on our sources: Where possible, we rely on the work of established teams, organisations and academic literature (e.g. reports from McKinsey, media houses like Reuters, academic institutions like MIT or Stanford, or established nonprofits like Our World In Data). However, since the AI ecosystem is moving very quickly, sometimes the best available evidence consists of insider information published in the form of blog posts or media announcements where the underlying data are harder to verify. In order for this report to be timely, we included these sources as well but tried not to rely on them too heavily and always searched for more sources. Throughout this report, we try to avoid speculation as much as possible and present the neutral data we found, with an interpretation of what it all means at the end of the Conclusion section.
Introduction
Artificial intelligence has emerged as a dominant technological narrative, appearing prominently in policy discussions, media coverage, and corporate strategies. The discourse around AI presents contrasting viewpoints: proponents highlight breakthrough capabilities in scientific research, enterprise productivity, and creative applications, while critics point to performance inconsistencies, reliability issues, and potentially exaggerated timelines. This divergence in perspectives creates a complex landscape for decision-makers seeking to understand AI’s current capabilities and future trajectory. A structured, evidence-based examination can help navigate between these positions, providing clarity on where meaningful progress exists and where uncertainty remains.
The debate is not just an academic one. AI’s rise is already reshaping how governments, businesses, and individuals approach the future. Policymakers are crafting laws to regulate its development and deployment, tech companies are investing billions into new AI capabilities, and citizens are grappling with how AI might disrupt their jobs and daily lives.
This report takes a pragmatic, evidence-led approach to that question—moving past the polarised headlines to explore what’s real, what’s overblown, and what remains uncertain.
By grounding our analysis in empirical evidence—from capability benchmarks and adoption rates to expert timelines and investment flows—we aim to give a balanced view of AI’s progress and promise. We explore areas where progress is already meaningful, such as scientific discovery and enterprise productivity, and highlight the structural challenges—like reliability, resource constraints, and social risks—that complicate forecasts of transformation.
In doing so, this report supports policymakers, researchers, and other decision-makers in navigating AI’s trajectory with nuance rather than falling for extremes.
AI’s global surge—How much attention and resources is AI receiving and is it generating returns?
Attention
There is growing public and policy attention on artificial intelligence. The frequency of Google searches for ‘AI’ has increased 10x over the past 5 years[14], and the number of news articles has risen significantly since 2015[15], with a particularly sharp increase in coverage[16] following ChatGPT’s release in late 2022.
AI is also getting a lot of attention in the world of policymaking. Mentions of AI in legislative proceedings[17] across the globe have nearly doubled, rising from 1,247 in 2022 to 2,175 in 2023. AI was mentioned in the legislative proceedings of 49 countries in 2023. Moreover, at least one country from every continent discussed AI in 2023, underscoring the truly global reach of AI policy discourse.
Looking at public opinion tracked by the Ipsos 2024 survey[18], the proportion of those who think AI will dramatically affect their lives in the next three to five years is now 66%. Moreover, 50% of people say that AI products have already profoundly changed their lives in the past 3-5 years. Many people also use AI products on a daily basis: ChatGPT, which represents 70% of the market share[19] of AI tools, has 400 million weekly users[20], already making it one of the largest consumer products on the internet. Overall, in February 2024, 23% of US adults claimed to have ever used ChatGPT.[21]
However, expectations are mixed. While the majority of respondents (66%) to the Ipsos 2024 global survey[22] think AI will dramatically affect their lives in the next three to five years, and 50% already reported such change in the past 3-5 years, when zooming in on economic expectations, people are mostly sceptical. Only 37% of respondents feel AI will improve their job, 36% anticipate AI will boost the economy, and 31% believe it will enhance the job market. Meanwhile, the majority of respondents (60%) think AI will change how they do their job, but not replace them altogether in the next 5 years. Moreover, there is a growing nervousness toward AI products and services for 50% people, up 13% from 2022[23].
When zooming in on specific countries, perceptions of AI’s benefits versus drawbacks vary considerably by country, according to the Ipsos survey[24]. 83% of Chinese, 80% of Indonesian, and 77% of Thai respondents view AI products and services as more beneficial than harmful. In contrast, only 39% of Americans agree with this perspective and Belgian (38%) and Dutch (36%) respondents are even more sceptical. Among the 32 countries surveyed, the Anglosphere and European countries exhibited the most scepticism.
Investment
AI is also getting a lot of investment. Despite a decline in overall AI private investment, funding for generative AI surged,[25] growing nearly 8 times from 2022 to reach $25.2 billion in 2023. Major players in the generative AI space, including OpenAI, Anthropic, Hugging Face, and Inflection, reported substantial fundraising rounds. The Stanford AI Index Report of 2024 notes ‘generative AI’ to have accounted for over a quarter of all AI-related private investment in 2023.
Funding for generative AI surged[26], growing nearly 8 times from 2022 to reach $25.2 billion in 2023 |
Out of $95.99 billion private investments in all AI in 2023[27], most investments happened in the US ($67 billion) and was roughly 8.7 times greater than the amount invested in the next highest country, China ($7.8 billion), and 17.8 times the amount invested in the United Kingdom ($3.8 billion). The data suggests that the gap in private investments between the United States and other regions is widening over time. That holds for generative AI in particular, where the combined investments of the European Union and United Kingdom in 2023 ($0.74 billion) stagnated, while the U.S. sharply increased its investment to $22.46 billion.
And these investments do not seem to be tapering off any time soon. In the first three quarters of 2024[28] Microsoft, Meta, Alphabet and Amazon spent nearly $170 billion on AI, up 56% from the same fraction of 2023. Big Tech’s AI spending continues to accelerate at a blistering pace, with the four giants well on track to spend upwards of $250 billion predominantly towards AI infrastructure in 2025. Alongside this, other investors led by OpenAI, SoftBank, Oracle and MGX announced a plan coined Stargate[29] to invest $100 billion yearly up to a total of $500 billion over the next four years to build new data centres for AI, supported by the Trump administration.
Other global actors have responded to these significant US investments by announcing their own funds: China with fully state-sponsored one trillion Yuan[30] (approximately $138 billion) “AI Industry Development Action Plan” and EU with a total of over €200 billion[31], consisting of €150 billion privately funded EU AI Champions initiative and €50 billion InvestAI initiative in large part funded from public sources, mostly to build public computing infrastructure and replicate the success story of CERN.
Returns
These investments are also beginning to generate revenues: Microsoft is already approaching[32] $10 billion in AI revenues in 2024, and estimates[33] to see $100 Billion in AI revenue by 2027. Amazon and Alphabet have seen[34] AI revenue in the billions in 2024.
Looking at the level of concrete products, it’s often hard to find public information on costs and revenues and attribute them to specific products, but there are a couple of estimates we can use as very rough indicators. For example, estimates for GPT-4’s training cost range from $41 million (excluding salaries)[35] to over $100 million[36], while OpenAI projected $1.6 billion[37] in revenues in 2023 and $3.7 billion[38] in 2024, with approximately $700 million and $2.7 billion, respectively attributable to chatGPT[39] mostly powered by GPT4 models (since March 2023), putting the estimated revenue of GPT4 models at $3.4 billion. That would put the cost-to-revenue ratio at 1:34, however, the real ratio is probably lower since some of the product variations in GPT4 suite might have had substantial costs as well, and the cost of providing these models to customers is not included. At the level of whole company, OpenAI decided to reinvest all this revenue and fundraised significant additional funds from investors to create new generation of products and stay ahead of the competition, so even though they see significant revenue, their cash flow is negative and some estimate[40] they will see positive cash flows only as late as in 2029.
Finally, costs of providing AI services shows unprecedented speed of depreciation. According to Dane Vahey from OpenAI, it took only 18 months for AI models to drop in price by 99%[41], whereas it took solar energy around 40 years[42] to show the same drop in price, making AI one of the fastest cost-depreciating technologies ever invented (see Figure 1). If these industry reported figures are accurate, this rapid cost depreciation suggests the technology becomes dramatically more accessible over time, unlocking potential for adoption and real-world impact beyond the current hype.
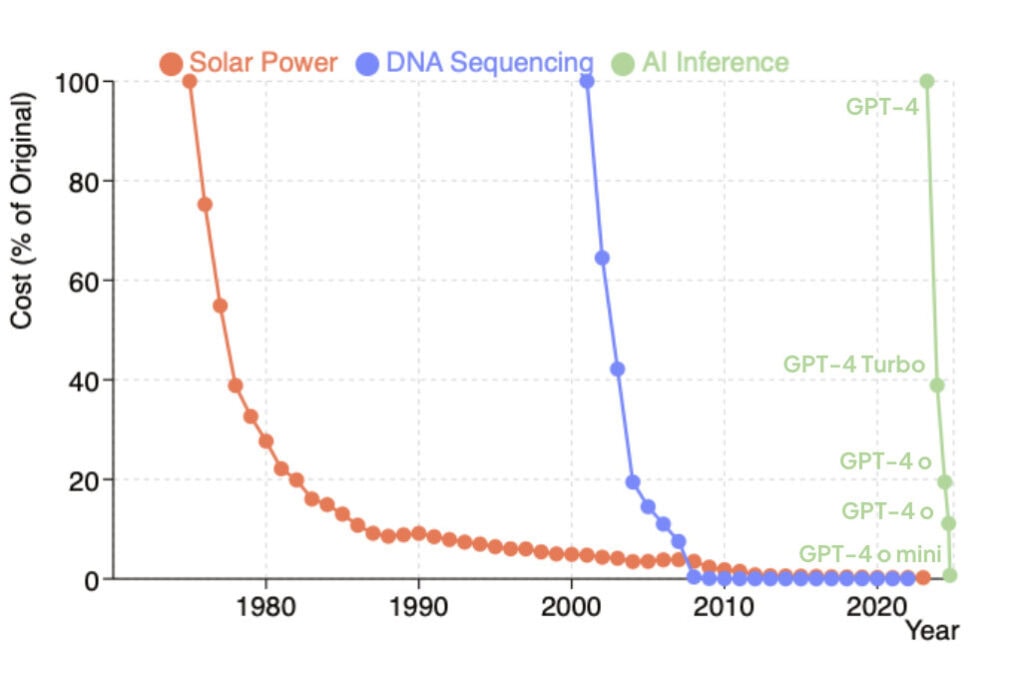
We’ve seen that AI is receiving a lot of attention from the general public, media and policy-makers, as well as significant levels of investment and infrastructure build-out, while becoming more accessible very fast. But what is the transformative potential of AI? We will explore this question in the next chapter, starting from mapping where the AI capabilities really are today.
Summary: AI has attracted wide public attention and significant resources, creating early revenue streams while still requiring large investments. Media coverage and Google searches for AI have increased tenfold in five years, while legislative proceedings mentioning AI have nearly doubled since 2022. Investment flows tell a similar story, with tech giants committing hundreds of billions to AI infrastructure, and governments launching massive funding initiatives. While large companies like Microsoft are beginning to see significant revenue from AI products, perhaps most remarkable is how quickly costs are reportedly falling—99% in just 18 months, a pace that took solar power 40 years to achieve. This could mean we’re witnessing one of the fastest cost-depreciating technologies ever developed.
AI today—What is the current state of AI capabilities and societal impact?
In the previous chapter, we looked at how much resources in terms of attention and investments AI is getting and briefly looked into first impacts in terms of returns on investments. In this section, we will continue this focus on the state of AI today, breaking it down into mapping most recent AI capabilities, adoption rates, and actual impact. From there, we take a look at some of the future capabilities already looming on the horizon.
Capabilities
AI has a different set of strengths and weaknesses compared to humans[47]. AI is much faster (as much as 6000x at the time of publication of this analysis), able to absorb and operate with much larger amounts of information, good at repetitive tasks and always available. The general trend is that AI is growing in diverse capabilities and catching up, or even surpassing humans in growing numbers of specific skills. In the past few years, we have seen AI models surpassing human performance in image classification, visual reasoning, and English understanding (see Figure 10), yet it trailed behind on more complex tasks like competition-level mathematics, visual commonsense reasoning and planning. Developments in 2024 showed that AI is quickly catching up even in these more complex domains showing signs of advanced reasoning capabilities.
However, measuring AI capabilities accurately presents significant methodological challenges. AI companies often present progress on standardized technical benchmarks, which as critics note, may not translate well to messier real-world contexts where problems are less structured[48]. Moreover, many benchmarks can be gamed[49] by AI companies fine-tuning models specifically for benchmarks, or selectively reporting their best results. Fortunately, newer evaluation approaches, such as direct comparisons of AI with human experts in real-world tasks or analysing successful real-world deployments, or are yielding more convincing evidence of AI capabilities and progress.
AI performance is still high variance—very strong on some tasks humans often struggle with, yet surprisingly weak on other tasks humans find easy. Sceptics often point to the valleys of AI capabilities, such as AI models not being able to complete a relatively trivial task like counting the number of r’s in strawberry[50] or mistaking satirical content in the Onion for research-based advice[51]. While valleys can potentially create negative transformative impacts when misapplied at scale, many of the benefits and risks might come rather from the peaks—from people being able to utilise the AI models in ways where their capabilities are strongest— because AI could selectively be used where their capabilities surpass those of humans (e.g. protein folding prediction) leading to substantial reductions in required time and resources. In order to assess the potential for transformative impact, we should therefore look at the peak of capabilities rather than the valleys.
What are such peaks of AI capabilities currently?
Multimodality
A big update from 2024 is that frontier AI models are not just language models anymore—they can handle text, code, images, audio, and even video and translate one to the other interchangeably. For example, a model can receive a photo of a plate of cookies and generate a written recipe as a response and vice versa. In the medical setting, this could in the future look like an AI model processing X-ray images, patient symptoms (text input), and audio descriptions from a doctor to generate a comprehensive diagnostic report, recommend further tests, and suggest potential treatments. During natural disasters, a model could analyse satellite imagery, social media posts (text, images, video), and audio emergency calls to identify areas most in need of rescue, optimise resource allocation, and predict the path of the disaster. In agriculture, models could analyse drone images of crop health, soil composition reports (text), and audio data from machinery to optimise irrigation, detect pest infestations, and suggest planting strategies. In the future, we might see additional modalities integrated like thermal imaging, 3D spatial data, motion and kinetic data, olfactory data, EMG signals, etc… unlocking many new applications.
Mathematics
- AlphaGeometry2, a special model tailored to solving mathematical questions, has surpassed an average gold medalist in solving International Math Olympiad geometry problems[52].
- OpenAI’s o3 model has demonstrated a significant advancement in AI’s mathematical reasoning capabilities[53] by achieving a 25.2% success rate (up from 2% of previously-best models) on the FrontierMath[54] benchmark. This benchmark was put together by world-leading mathematicians and comprises hundreds of original, expert-level mathematics problems that typically require hours or even days for specialist mathematicians to solve. The significance of this achievement extends beyond mere problem-solving. Mathematics requires extended chains of precise reasoning, with each step building exactly on what came before. The ability of AI to engage in such complex reasoning tasks suggests promising applications in various scientific and engineering domains, where advanced problem-solving skills are essential.
Biology and Chemistry
- Deepmind’s AlphaFold, has significantly outperformed human experts in predicting protein structures[55]. In the 14th Critical Assessment of Structure Prediction (CASP14) competition, AlphaFold achieved a median Global Distance Test (GDT) score of 92.4 out of 100 (with the second best scoring 75), indicating predictions nearly identical to experimentally determined structures. This performance surpassed all other participants, including human teams, marking a substantial leap in the field. The impact of AlphaFold is profound, facilitating accelerated drug discovery, enhancing our understanding of diseases, and contributing to the development of novel enzymes for industrial applications. In recognition of this achievement, Demis Hassabis and John Jumper of DeepMind, along with biochemist David Baker, were awarded the 2024 Nobel Prize in Chemistry[56] (see Figure 2).
- Looking at more general models, OpenAI’s o3 achieves 87.7% on GPQA Diamond, a set of graduate-level biology, physics, and chemistry questions[57]. Human experts who have or are pursuing PhDs in the corresponding domains reach 65% accuracy[58] (74% when discounting clear mistakes the experts identified in retrospect), while highly skilled non-expert validators only reach 34% accuracy, despite spending on average over 30 minutes with unrestricted access to the web (i.e., the questions are “Google-proof”). This suggests that current frontier general models are performing better than PhD-level experts.
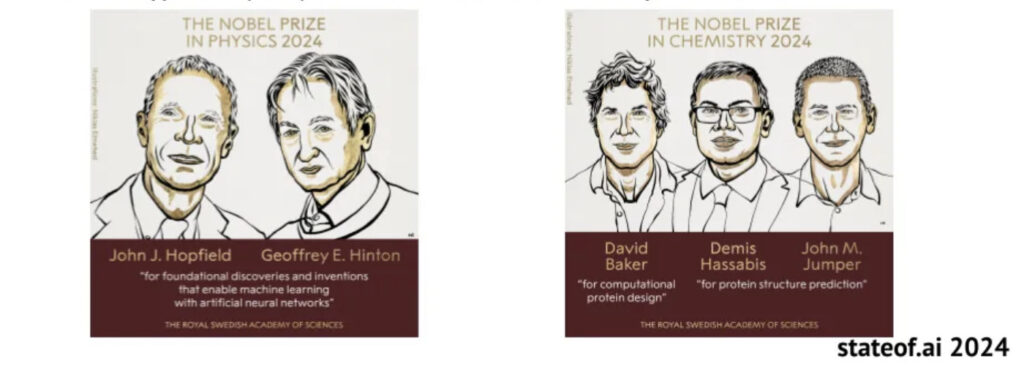
Medicine
- In an experiment, doctors who were given ChatGPT to diagnose illness[62] did only slightly better than doctors who did not. But the chatbot alone outperformed all the doctors. ChatGPT scored an average of 90% when diagnosing a medical condition from a case report and explaining its reasoning. Doctors randomly assigned to use the chatbot got an average score of 76%. Those randomly assigned not to use it had an average score of 74%. Another study[63] found that compared to the physicians, GPT‐4 using only text input achieved a significantly better diagnostic accuracy for the common clinical scenarios in its top 3 suggestions for diagnoses (84.3% vs 100%) and a better diagnostic accuracy for the most challenging cases in its top 6 suggestions for diagnosis (49.1% vs 61.1%), and similar results could be seen in other studies[64] as well.
- Another study found ChatGPT to be nearly 72% accurate across all medical specialties[65] and phases of clinical care including generating possible diagnoses and care management decisions, and 77% accurate in making final diagnoses.
- In the largest randomised controlled trial of medical AI as of February 2025, doctors assisted with AI were able to detect cancer in 29% more cases[66] with no increase in false positives, all the while reducing radiologists’ workload.
- Researchers at the University of Pennsylvania have employed AI to expedite antibiotic discovery[67], reducing the timeline from years to mere hours[68]. Their AI-driven methods have already yielded numerous preclinical candidates, showcasing the transformative potential of AI in antimicrobial research. Additionally, AI has been instrumental in identifying new antibiotics effective against resistant bacteria[69].
Engineering and Design
- Code Generation: OpenAI’s o3 model ranked among the top 175 best coders in competitive programming[70], outperforming approximately 99.8% of human participants. The o3 model also achieved an accuracy of 71.7% on SWE-Bench Verified, marking a substantial improvement over its predecessor scoring 48.9%, which signifies a notable leap towards AI systems capable of handling real-world software development tasks with proficiency comparable to human experts. AI-driven code generation tools, such as GitHub Copilot, have become integral to modern software development. Copilot assists developers by providing code suggestions and autocompletions, effectively acting as an AI pair programmer.
- Material Science: AI-driven simulations have led to the discovery of new alloys with enhanced properties[71]. Researchers at MIT have utilised machine learning to design high-performance aluminum alloys, achieving a balance between strength and thermal conductivity. This approach accelerates the development of materials suitable for aerospace and construction applications and follows up on previous uses of AI to accelerate the materials discovery process[72] of stable inorganic materials, providing a vast database for researchers to explore materials with desirable properties for various applications, such as in electronics, catalysis and energy storage[73].
General Reasoning
- Another breakthrough came in the ARC-AGI benchmark, which tests a model’s ability to reason in ways that general intelligence would require[74]. It is designed to evaluate an AI system’s ability to handle novel tasks and demonstrate fluid intelligence, requiring understanding of basic concepts such as objects, boundaries, and spatial relationships[75]. OpenAI’s o3 achieved a score[76] of 75.7% (and even 87.5% when some restrictions were relaxed), showcasing a large progress in making AI models more generalist. As a comparison, it took 4 years to go from 0% with GPT-3 in 2020 to 5% in 2024 with GPT-4o. One study[77] also shows that the human average score on this benchmark is only 64.2%, suggesting that AI models are now able to generalise better than humans. The benchmark’s creator and well-known sceptic of the current AI paradigm François Chollet[78] said during the live streaming of o3: “When I see these results, I need to switch my worldview about what AI can do and what it is capable of.”
- On another measure of reasoning abilities, benchmark MMMU, we can also see a sharp increase in performance during 2024. While the best model in 2023 scored 59.4%, the best recorder score of 2024 is 78.2%[79], closely approaching the medium human expert baseline (82.6%). This best performance was made by OpenAI’s o1 model predecessor of the more advanced o3 model for which we don’t have results on this benchmark yet, but given other results, it is likely that the human baseline was exceeded.
Art and creativity
- Recent studies have found that readers often prefer AI-generated poetry over human-authored works[80]. In blind tests, participants rated AI poems higher in qualities such as rhythm and beauty, and frequently mistaking them for human creations.
- Humans also struggle to distinguish real photos from AI-generated ones, with a misclassification rate of 38.7%[81]. This highlights the sophistication of AI in creating photorealistic images that can deceive human perception.
- In 2024, the AI-generated song “Verknallt in einen Talahon” by Austrian producer Butterbro became the first of its kind to enter the German Top 50 charts, debuting at number 48[82]. This achievement underscores AI’s growing influence in music composition and its ability to produce commercially successful content.
Adoption
History tells us that adoption of technology is the most important factor[83] for their economic impact. All these AI capabilities are one thing, but what good are they if no one uses them? Next, we examine this point further: How many people actually use AI systems? What is the state of AI adoption?
Businesses
Eurostat data[84] from the National Statistical Authorities collected over the first few months of 2024 suggest relatively low levels of AI adoption: only 13.5% across all enterprises in the EU (see Figure 3), though still a significant increase from 8% in 2023. It further showed that AI is used in some economic activities a lot more than in others.
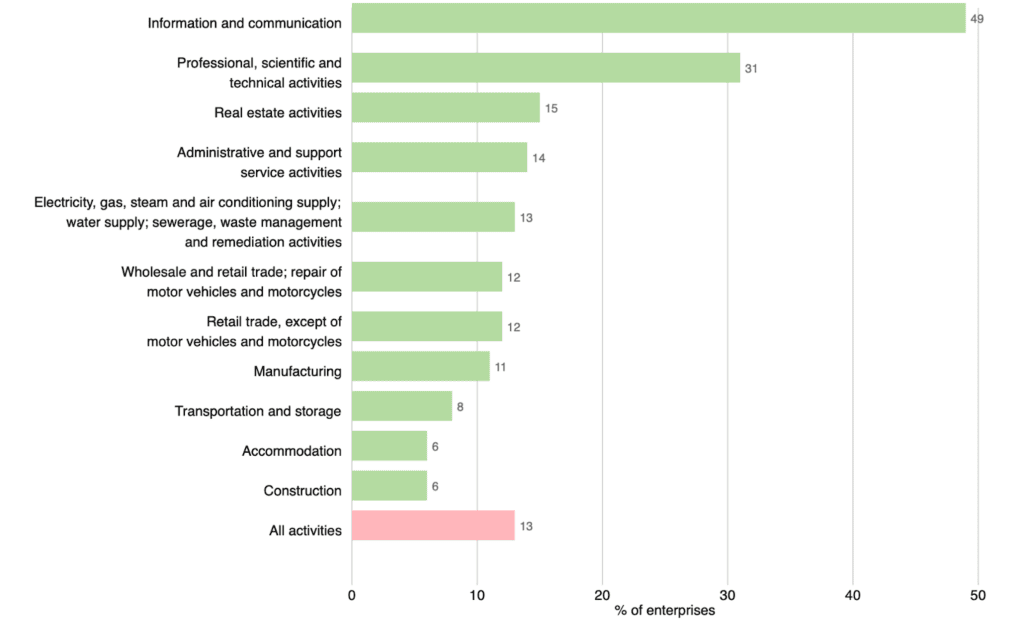
Interestingly, this is a very different finding to other private surveys that collected data towards the end of 2023. For example, the survey from February 2024 conducted by Strand Partners[86] of over 16,000 citizens and 14,000 businesses across the European Union, the UK, and Switzerland found that 33% of businesses in the EU have adopted AI in 2023, compared with 25% in 2022. It also found that 38% of companies were experimenting with AI.
Deloitte surveyed[87] 30,252 consumers and employees in Belgium, France, Germany, Ireland, Italy, Poland, Spain, Sweden, Switzerland, the Netherlands, and the United Kingdom in Summer 2024. Of these respondents, 44% had used gen AI, 22% had not used it but were aware of it, and 34% were either unaware or unsure of any gen AI tools. Among those familiar with gen AI, nearly half (47%) have used it for personal tasks, while only a quarter (23%) said they have used it for work. Further, 65% of European business leaders confirmed that they are increasing their investments in gen AI due to the substantial value realised so far.
The latest McKinsey Global Survey[88] on AI from May 2024 found that 65% of respondents reported that their organisations are regularly using generative AI, nearly double the percentage from their previous survey just ten months ago (see Figure 4).
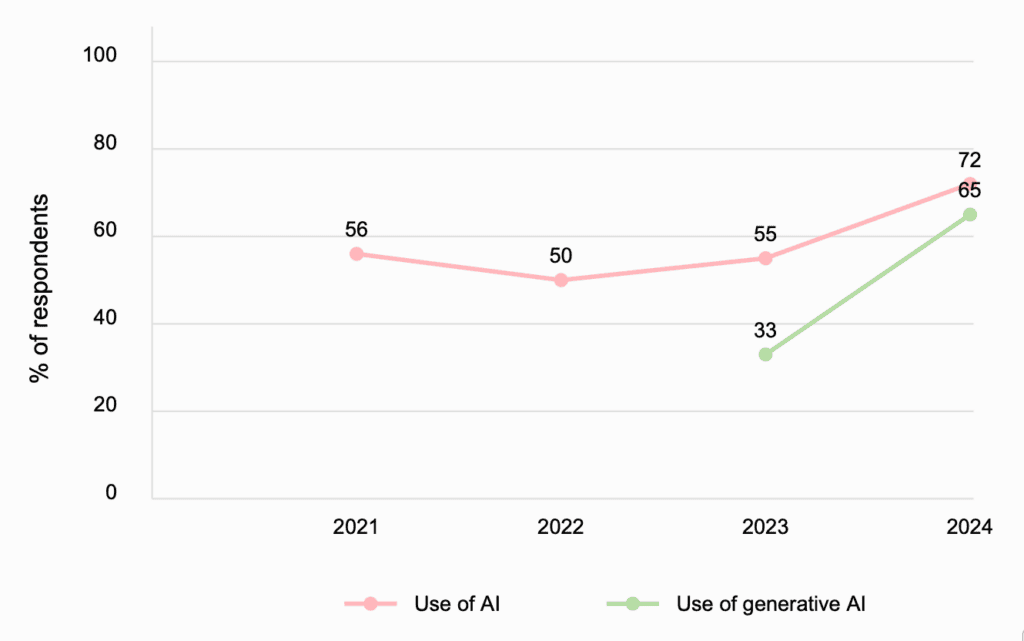
Yet a recent Pew Research survey indicates that the situation in the general public seems to be different—only about a third of U.S. adults have ever used an AI chatbot[90]. This points to a more cautious or limited uptake among the broader public compared to reported within-organisations adoption figures, highlighting that while many organisations are forging ahead, mainstream adoption is still emerging, varying considerably by context and user needs.
How do people use AI?
According to a Deloitte survey[91], people use AI mainly for searching and gathering information, generating ideas, and creating or editing content. In both personal and professional settings, AI-powered tools help summarise text and translate languages. In the workplace, many employees see AI as a way to make their jobs easier and more enjoyable while also improving their skills and career prospects. Despite some concerns around governance and regulations, cultural resistance to AI adoption appears to be relatively low according to European leaders surveyed in the latest Deloitte’s State of Generative AI in the Enterprise Q3 survey[92].
Responses to the 2024 McKinsey survey[93] also suggest that companies now use AI in more parts of the business. Half of respondents say their organisations have adopted AI in two or more business functions, up from less than a third of respondents in 2023. The average organisation using gen AI is doing so in two functions, most often in marketing and sales and in product and service development, as well as in IT.
Globally, AI adoption is growing very fast, perhaps faster than any other technology in the past (see Figure 5 for a visual comparison), although still at a very early stage. After its launch, ChatGPT became the fastest growing app in history[94], outpacing even the viral social media launches of Instagram and TikTok. Currently, leading gen AI app ChatGPT has 400 million weekly users[95], already making it one of the largest consumer products on the internet. Since its release in 2023, 23% of US adults claim to have ever used ChatGPT[96] as of February 2024.
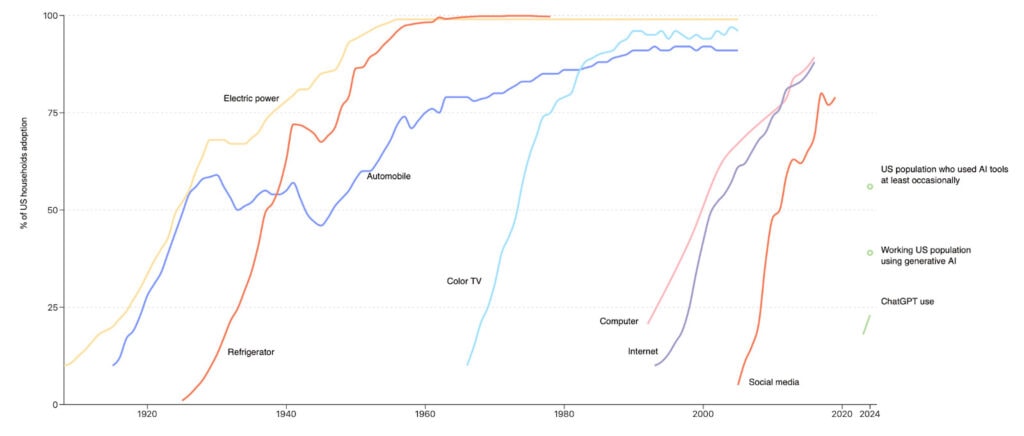
Impacts
We have seen that AI has some advanced capabilities already and that increasingly many people and businesses use it—but does it actually produce any change in the real world? What are the actual impacts of AI that we have seen so far?
Economic impacts
The latest McKinsey Global Survey[101] on AI from May 2024 found that a substantial fraction of organisations are already reporting material benefits from ‘generative AI’ use, both in terms of cost decreases and revenue growth in the business units deploying the technology. On average 39% of respondents report some kind of cost reduction and 44% report revenue increases, across all organisational functions (see Figure 6).
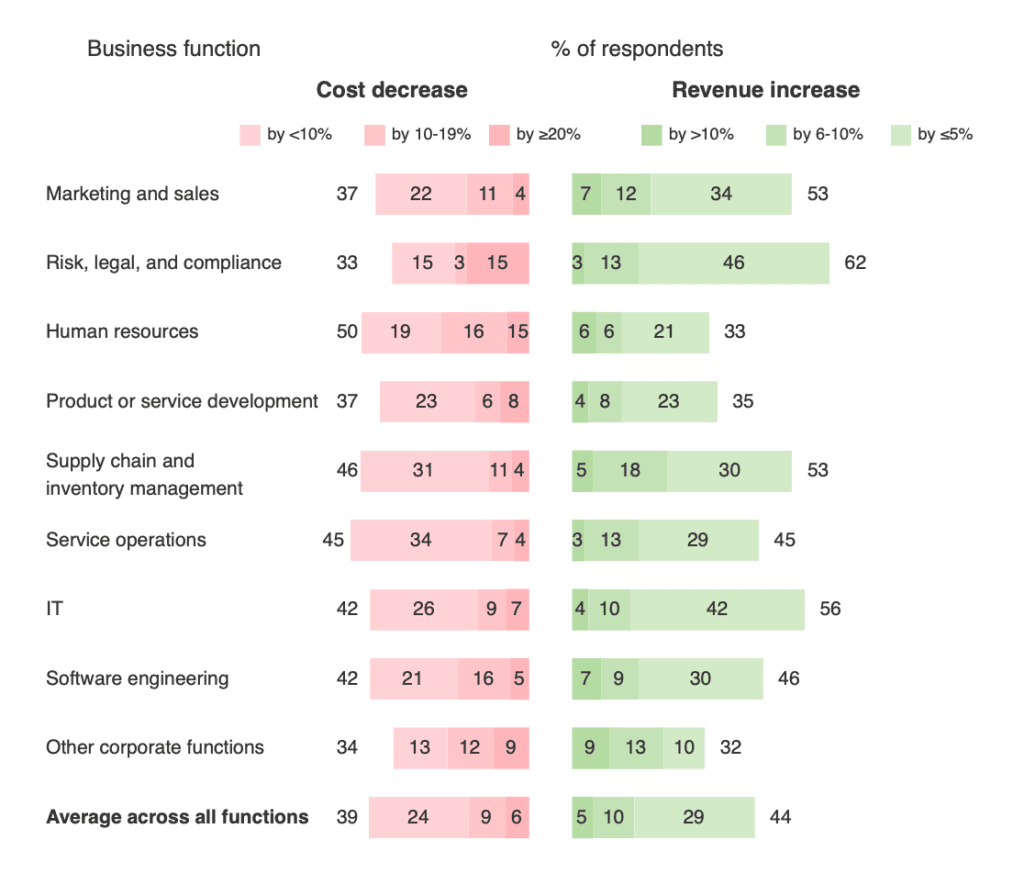
Google CEO Sundar Pichai claimed that AI tools now generate over 25% of the code at Google[103], while an Amazon executive[104] reports that the company has saved “the equivalent of 4,500 developer-years of work” and “an estimated $260 million in annualised efficiency gains.”
Increases in productivity
One of the most often mentioned benefits of AI is its capacity to help workers increase their productivity. Recent general-purpose AI systems already provide substantial productivity gains, with claims of an average 25% productivity increase[105] by Goldman Sachs. A meta-review[106] by Microsoft focusing on coding, which aggregated studies comparing the performance of workers using Microsoft Copilot or GitHub’s Copilot—LLM-based productivity-enhancing tools—with those who did not, found that Copilot users completed tasks in 26% to 73% less time than their counterparts without AI access. Looking at different “softer” sectors, a Harvard Business School study[107] revealed that consultants with access to GPT-4 increased their productivity on a selection of consulting tasks by 12.2%, speed by 25.1%, and quality by 40.0%, compared to a control group without AI access. Likewise, a National Bureau of Economic Research paper[108] reported that call-centre agents using AI handled 14.2% more calls per hour than those not using AI. Finally, a study[109] on the impact of AI in legal analysis showed that teams with GPT-4 access significantly improved in efficiency and achieved notable quality improvements in various legal tasks, especially contract drafting.
Accelerating scientific discovery
Moreover, generative AI systems seem capable of accelerating scientific discovery by streamlining ideation and prioritisation workflows. For example, a 2024 study from MIT[110] found that material scientists assisted by AI systems “discover 44% more materials, resulting in a 39% increase in patent filings and a 17% rise in downstream product innovation.”
As the OECD[111] noted in 2023, “accelerating the productivity of research could be the most economically and socially valuable of all the uses of artificial intelligence”. However, it is difficult to ascribe societal or economic value to any specific AI-assisted scientific research so soon after the technology has been adopted at scale, if at all[112], so we currently lack hard evidence for knock-on impacts in this area.
Summary: Current AI systems demonstrate remarkable but uneven capabilities. In specific domains, frontier models have surpassed human experts—solving graduate-level scientific problems, predicting protein structures, achieving gold-medalist performance in mathematics olympiads, and even outperforming doctors in medical diagnosis—while still struggling with seemingly simple tasks. Adoption is accelerating faster than any previous technology, with business use doubling year-over-year in some sectors and 23% of US adults having used tools like ChatGPT. Early impact measurements show promise: organizations report both cost reductions and revenue increases from AI implementation, productivity gains range from 12-40% across knowledge work domains, and scientific discovery is being meaningfully accelerated, suggesting that despite reliability concerns, AI is already creating real-world value beyond the hype. |
AI trajectories—How soon could AI become transformative?
In the previous chapters, we concluded that AI does receive a lot of attention and resources, it has already reached some impressive capabilities, while being increasingly quickly adopted, and we can see some early signs of impacts. But the pressing question remains: When will the transformative impacts of AI arrive? Do we have a couple decades to prepare, or rather a couple of years? To answer this, we start by looking to the horizon for emerging capabilities that are likely to become reality very soon, after which we zoom out to see a bigger picture of AI’s past progress, potential obstacles and expert forecasts.
What are the most important AI advancements on the horizon?
In previous sections, we have focused on already materialised AI capabilities, adoption and impacts. In this section we will set our sight on the horizon, to see the contours of what might come in the next few years. What are the most important emerging capabilities most likely to be developed in the near future?
Autonomous agents
Many business leaders[113] and top AI companies[114] expect the future developments to go in the direction of creating more autonomous AI systems, that some call agents.
Recent advances in large language models have sparked serious momentum behind ‘AI agents’—systems that pursue goals independently, often in complex environments. Developers are already building early prototypes that can book flights, order takeaway, or populate a CRM system without human micromanagement. These agents combine an LLM with “scaffolding” software that lets them browse the web, execute code, or interact with apps and services. Though still error-prone, some companies are aiming far higher: envisioning agents that could autonomously manage a factory, conduct a cyber operation, or even run a business end-to-end. A 2024 workshop hosted by Georgetown’s CSET provides an overview of this situation and highlights the uncertainty around how quickly AI agents will mature—whether they’ll soon become widely deployed and highly capable, or remain niche research tools for the foreseeable future—underscoring the need for policy approaches that are flexible enough to accommodate either trajectory[115]. A few months later, new research from METR finds that the length of tasks AI agents can handle is doubling roughly every seven months[116]—pointing to a kind of “Moore’s Law” for autonomous capability. Taken together, these expert insights offer a grounded glimpse into what AI agents might realistically enable, and underline the need for thoughtful preparation and oversight.
The evolution from current large language models like ChatGPT to more “agentic” AI systems would signify a shift from passive text generation to autonomous decision-making and action execution. While LLMs excel at understanding and generating human-like language, they typically require human prompts to operate and lack the capability to perform tasks independently. In contrast, agentic AI systems would possess autonomy[117], adaptability, and contextual awareness, enabling them to set goals, plan workflows, make nuanced decisions, and adjust to changing circumstances without continuous human oversight. For a practical example of how AI agents might work, check out this demonstration[118]:
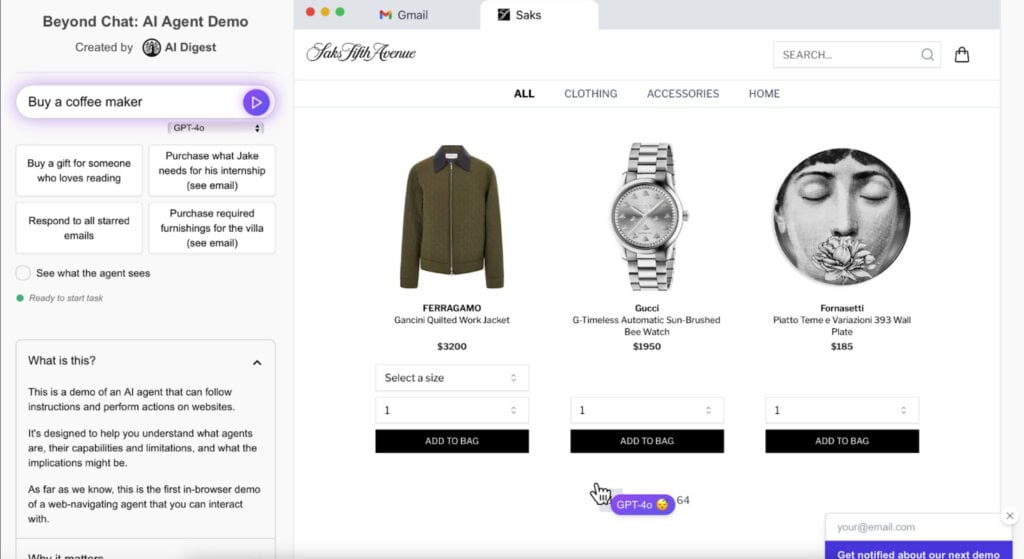
What could greater AI autonomy unlock?
One of the main effects predicted by many business leaders is accelerating large scale job displacement. Once AI models achieve a certain level of autonomy and understanding of the context they are operating in, they might become a preferable choice for employers due to their other strengths[119]:
- Speed. The fastest LLM produces output at 6000x the speed a human can[120];
- Scale. The number of AI agents deployed could surpass the human population, provided sufficient computational resources are available.
- Parallelisation. Tasks can be divided among thousands or even millions of AI agents.
- Cost of labour. Over time, agents become cheaper than human labour, especially when scaled. Right now we can get a proto-agentic system to perform a meta-analysis of 200 ArXiv papers for ~1% of the human cost. AlphaFold predicted 200 million protein structures, each of which would traditionally cost $100,000[121] and an entire PhD to determine. Further, transaction costs might also be much lower than for humans—hiring an AI agent will likely be much quicker and less risky than hiring a human worker, while not requiring the same levels of benefits and time off.
These kinds of advantages—speed, scalability, lower costs—have driven major shifts in labour before. One of the clearest historical parallels is the transformation of agriculture. In the late 18th century, around 90% of the American workforce was employed in farming; today, it’s only about 1%[122]. Yet over that period, food production didn’t just hold steady—it soared. From 1948 to 2017, U.S. agricultural output nearly tripled, even as labour inputs dropped by more than 80%[123]. Mechanisation, chemical inputs, and more recently, data-driven precision farming, meant that a much smaller group of workers could produce far more than was once possible. It’s a striking example of how technological progress can unlock massive productivity gains while dramatically reshaping the workforce, though at the cost of personal downsides to many, like during any period of labour disruption. The difference now is pace: what took agriculture centuries, AI might do across many sectors in just years or decades.
In addition to benefits that could be unlocked, AI agents may also exacerbate a range of existing AI-related issues as well as create new challenges. For instance, the ability of agents to pursue complex goals without human intervention could lead to more serious accidents; facilitate misuse by scammers, cybercriminals, and others; and create new challenges in allocating responsibility when harms materialise. Additionally, there is growing evidence that frequent reliance on AI tools may erode human skills over time. A 2024 study in Cognitive Research: Principles and Implications found that using AI assistants can accelerate skill decay in experts and hinder novices in developing competence—often without users realising, as the AI creates a false sense of mastery[124]. A well-known parallel is aviation: pilots became less proficient at manual flying due to automation complacency, prompting U.S. regulators to recommend regular hands-on training[125]. More recent studies have found that heavy AI use is associated with weaker critical thinking skills, likely due to “cognitive offloading,” where users defer too much of the mental effort to the system[126]. This effect is especially pronounced in younger users, raising concerns about long-term impacts on learning and problem-solving. These findings highlight a concrete risk: as AI systems take on more cognitive tasks, humans may lose the ability—and the habit—of doing that work themselves. Finally, the potentially faster pace of change inherently heightens the risks of various short-term pains.
Where is the industry now?
The current state of AI agents represents a rapidly evolving frontier, with clear signs of progress and limitations. Although many agentic systems remain rudimentary, significant investment and development by leading AI companies suggest that more capable agents may soon enter the workforce, likely revolutionising specific industries before achieving widespread general-purpose utility.
CEO of Nvidia Jensen Huang recently predicted[127] that we will see AI agents first in software engineering, digital marketing, and customer service. A recent report by the Center for Security and Emerging Technology (CSET) estimates[128] that the rapid adoption of narrow-purpose agents could begin scaling within 2–3 years initially in industries like logistics, customer support, and software development, but broader adoption in more sensitive fields (e.g., legal, medical, or strategic decision-making) may take longer due to trust, safety, and governance challenges.
The uptake of AI agents will likely vary by domain, driven by technical feasibility, economic incentives, and regulatory constraints. According to both Huang and the CSET report, early adoption is likely in industries where:
- Tasks are repetitive and rule-based: Sectors like customer service, logistics, and sales are ripe for rapid adoption because agents can operate effectively in structured environments with clear objectives.
- High data availability exists: Domains such as marketing, cybersecurity, and software engineering offer robust feedback loops and data for agent fine-tuning, accelerating deployment.
- Regulatory barriers are lower: Companies are likely to first deploy AI agents in domains with minimal regulatory oversight, avoiding sectors like healthcare or financial advisory where compliance and liability risks are higher.
While previous AI systems like AlphaZero succeeded in closed, rule-defined environments like chess, Go, and shogi, they struggled in more dynamic settings, lacking the ability to continuously learn. This obstacle seems to be to a large extent overcome by Voyager[129], a GPT-4-based agent that can explore, plan, and learn in virtual 3D open-ended worlds.
OpenAI has launched[130] its “Operator” agent designed to manage tasks such as scheduling meetings, coordinating with various stakeholders, and handling follow-up communications without human oversight, streamlining administrative workflows. Anthropic has introduced[131] an experimental feature that allows its AI model to observe a user’s screen, move the mouse cursor, click buttons, and fill out forms, automating computer tasks and executing online transactions like ordering pizza. Google DeepMind recently introduced[132] agentic features that can operate from a browser and focuses on interoperability with other applications from Google or external.
Looking at the more formal measurements of progress, there is not much information on scores of the latest as-yet-unreleased models on agentic capability benchmarks or other assessment methods. However, looking at the progress from 2022 to 2023, we have seen almost doubling of the best score on AgentBench[133], a new benchmark designed for evaluating LLM-based agents. Another benchmark, PlanBench[134], measures the ability of AI models to develop plans, one of the important ingredients of autonomous agency. According to recent study, OpenAI’s o1-preview model achieved significant improvement in this ability[135] (between 27-97%, depending on the difficulty of the subtest), though it is still far from human baselines. One of the few indications about the latest models is Deepmind’s Project Mariner achieving a state-of-the-art result of 83.5%[136] working as a single agent setup in the WebVoyager benchmark[137], up from a best result of the 55.7% best result in January 2024.
While it is too soon to say whether these will become the ‘killer apps’ the industry hopes for, it is certainly evident that the main players in the AI sector are betting big on agentic AI.
Becoming generalist
We can also expect large models to become ever more generalist in their skillset. What once required custom-built models is increasingly accomplished by general-purpose systems straight out of the box. We’re already seeing this shift in practice: from catching software bugs[138] to translating languages[139] and diagnosing medical conditions[140], general-purpose models are matching or surpassing their specialised counterparts. This trend—which has been going on for several years now[141]—will likely continue as advancements in AI architectures, economic efficiency, and the rapid pace of innovation converge, making generalist systems the future of AI[142]. Generalist multimodal models are now capable[143] of processing and generating multiple data types—text, images, audio, and more—within a single framework. Moreover, researchers are using ChatGPT-like large language models in robotics[144], underscoring their versatility.
The ability to integrate diverse data streams makes these models more adaptable, efficient, and capable of handling complex, multi-domain challenges. Including more diverse data into the training process might also improve the overall performance of a model, solving potential data bottlenecks in some domains.
Building and maintaining specialised models for every application is also resource-intensive and costly. General-purpose models offer a scalable alternative, reducing development and operational costs while increasing flexibility. Organisations can deploy a single AI system across multiple functions, from customer service to predictive analytics, without needing extensive customisation. As AI models become more generalist, we can anticipate a future where a single system can seamlessly transition between tasks—whether drafting a legal document, composing music, or providing medical advice—without the need for significant task-specific customisation.
Where is the industry now?
One of the more formal measures of how much AI models can generalise and efficiently acquire new skills is a benchmark ARC-AGI[145] created by a noted sceptic François Chollet. Recently, the o3 model by OpenAI achieved a score[146] of 75.7% (and even 87.5% when some restrictions were relaxed), showcasing a large progress in making AI models more generalist. One study[147] also shows that the human average score on this benchmark is only 64.2%, suggesting that AI models are now able to generalise better than humans. According to many, the previous bottleneck was in visual reasoning[148] for large language models, but this bottleneck seems to have been resolved in some models. In Figure 7, you can see how fast this progress was achieved. Chollet said during the live streaming of o3: “When I see these results, I need to switch my worldview about what AI can do and what it is capable of.”
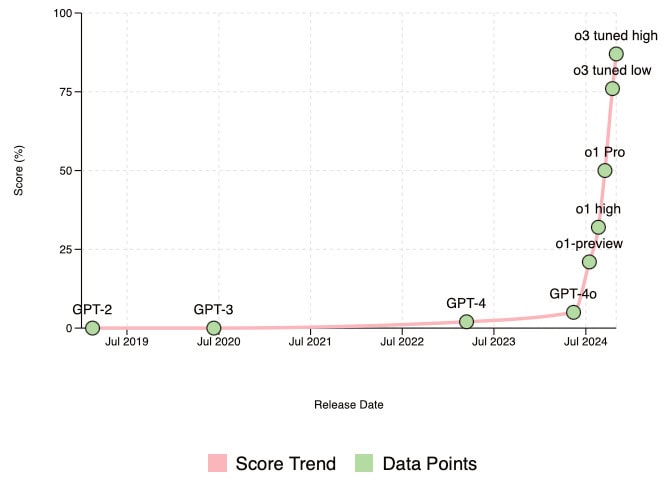
Automating AI R&D
Another emerging development worth increased attention is the ability of AI systems to develop new, more advanced AI models and automate the process of AI R&D. This would be the next step of the already self-reinforcing nature of AI progress (see Figure 8). While automating other parts of the economy could also bring about transformative changes, automating AI R&D specifically might have even starker ramifications. Once companies are able to automate the development process of the AI itself, we can get into a self-reinforcing loop where AI capabilities grow at blinding speed, without much chance of human interference. Some experts worry[150] that this is the point where AI gets out of our control, although it must be noted that some AI experts dismiss this concern.
AI R&D includes tasks like data collection and preprocessing, designing and training models, running experiments, analysing results, and fine-tuning algorithms. Automation in this field focuses heavily on engineering tasks like coding, debugging, and model optimisation, with tools like GitHub Copilot already enhancing productivity[151]. In a broader sense, this might also include automating the creation of inputs for AI models, such as chip design, data collection, and synthetic data generation. AI researchers anticipate that while tasks like hypothesis generation and experiment design will be harder to automate, AI will still accelerate its R&D, particularly in coding and debugging[152]. AI R&D is thus special compared to other industries and domains not only in its potential impact, but also in its feasibility – in large part it relies on coding and software development, capabilities in which current AI systems are already strongest[153]. Unlike other domains, AI R&D might not have natural bottlenecks like data availability or physical skill shortage slowing down the progress.
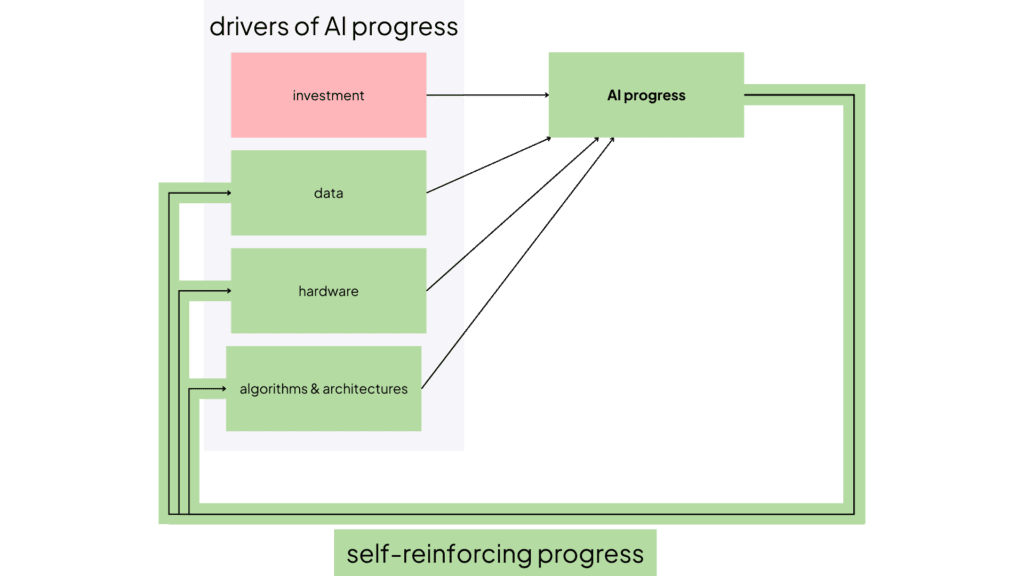
Source: CFG
Where is the industry now?
A recently created benchmark for measuring the extent to which frontier AI models can automate AI R&D[154], RE-Bench, shows that existing AI agents can outperform human experts in short-term, well-defined tasks—achieving up to four times higher scores with a two-hour time budget and generating solutions over 10 times faster—but fall behind in longer-term or more complex challenges, where humans achieve superior results. When both AIs and humans were given an eight-hour time budget, human performance kept increasing while AI performance plateaued, resulting in humans achieving slightly better scores than AI agents. When both were given 32 hours, humans significantly outperformed AI agents, achieving double the AI scores. While AI agents excel in rapid iteration and optimisation within constrained timeframes, their performance plateaus over extended periods, where humans continue to make meaningful progress, particularly in tasks requiring deeper contextual understanding and complex problem-solving strategies. This suggests that while AI systems are effective at accelerating specific types of R&D tasks, they are not yet capable of matching human adaptability and sustained productivity in challenging, open-ended scenarios. Notably, authors of these benchmarks used the most recent public models such as Claude-3.5-Sonnet and o1-preview.
However, even these shorter-term, lower complexity automation might already cause significant acceleration. For example, a recent study[155] found that today’s frontier AI models can already speed up typical AI R&D tasks by almost 2x.
On another benchmark for measuring AI research automation, MLE-bench[156], the o1 model’s output was on par with what would be expected from someone in the top 40% of human competitors with a score of 16.9% of the 75 tests. Another new benchmark for evaluating AI research agents’ performance, MLAgentBench[157], tests whether AI agents are capable of engaging in scientific experimentation—exactly the type of task where experts see the largest roadblocks[158]. More specifically, MLAgentBench assesses AI systems’ potential as computer science research assistants. The results demonstrate that although there is promise in AI research agents, performance varies significantly across tasks. While some agents achieved over 80% on tasks like improving a baseline paper classification model, all scored 0% on training a small language model. Note though that these tests are from 2023 so they only tested the previous generation of AI models (unlike the other benchmarks mentioned above), with no data available on the recent generation.
Finally, we can take a look at the predictions made by prediction markets and crowd forecasting platforms, which regularly[159] perform[160] better[161] than single experts and frequently equaling or exceeding the accuracy of expert groups thanks to aggregating diverse perspectives and combining insights from domain experts and informed generalists. According to the community on Manifold Markets, there is a 43% chance that AI will be recursively self-improving by mid-2026[162] and 31% chance that AI models will be able to do the work of an AI researcher/engineer before 2027[163]. According to the community on Metaculus, people predict that some form of full AI research automation[164] might appear in 2028.
Will these developments make AI transformative?
Having increasingly autonomous and generalist AI agents might already be sufficient to create transformative impact in our societies. On the benefit side, increasingly autonomous and generalist AI agents could dramatically accelerate scientific discovery and economic growth. For example, in medicine, they might identify novel drug candidates in days instead of years, while in renewable energy, they could optimise new battery technologies at an unprecedented pace.
On the risks side, such capabilities might displace millions of jobs, from administrative roles to highly skilled professions, creating societal upheaval. Additionally, their ability to act autonomously could inadvertently enable novel security threats, such as automated cyberattacks or misuse in developing advanced weapons, presenting risks we are currently ill-prepared to manage. The pace of change may outstrip our ability to adapt, narrowing the window for effective oversight and intervention.
In purely economic terms, AI’s ability to enhance both output and knowledge production may drive growth rates far beyond historical norms, driving us to the world of unprecedented change[165].
One capability that could multiply this acceleration and bring forward all transformative impacts much faster is automating AI research and development itself. If you feel like the pace of today’s world and speed of developing new AI capabilities is fast, automating AI R&D could make it much, much faster. Such capability would mean we don’t have to wait for (already very fast) groups of people to develop new AI capabilities, but that this process would happen in an automated manner, enabling parallelisation while not being slowed down by mundane needs like sleep or time for rest. Advancements that currently take years of coordinated human effort could unfold in weeks or even days. An automated AI R&D system might generate and test thousands of potential algorithms overnight, selecting and refining the best-performing ones. For instance, a fully automated AI lab could develop entirely new architectures tailored to specific tasks—like deciphering the origins of diseases or inventing materials for fusion energy—before researchers have even framed the question.
The economic ripple effects would be staggering. Economic[166] research[167] suggests that AI’s capacity to innovate and improve its own algorithms might lead to a self-reinforcing cycle of rapid technological advancement, further propelling economic expansion. For example, the rate of economic growth could surpass historical norms of 2-3% annually and rise to rates where global GDP doubles every few years, meaning that the growth acceleration could outpace even the most optimistic forecasts from the Industrial Revolution.
In such an environment, the risks also compound significantly.
Automated AI R&D could lead to a proliferation of systems that operate at levels of complexity beyond human understanding, increasing the likelihood of accidents and unintentional outcomes. For example, an AI system optimised for a specific task might inadvertently create cascading effects that destabilise critical infrastructure or other systems it interacts with. This aligns with findings in the International Scientific Report on the Safety of Advanced AI (p. 19)[168], which highlights the increased probability of “catastrophic accidents” as AI systems grow more autonomous and interconnected. The risk of losing control over such systems is particularly acute when they possess the ability to autonomously modify their own objectives or capabilities. Additionally, malicious actors could exploit these advanced capabilities to develop highly sophisticated cyberweapons or biologically engineered threats, pushing risks into domains where humans have limited or no capacity to intervene effectively. The accelerated pace of AI-driven innovation could outstrip our ability to manage these developments, raising the specter of a future where human oversight becomes inadequate or obsolete.
This future of automated AI R&D doesn’t just promise acceleration—it promises transformation on a scale we can barely comprehend. It demands urgent attention to safeguards, governance, and foresight to ensure humanity remains in control of the systems shaping its future.
Progress so far has been a rapid climb
Recent advancements in general-purpose AI have progressed at an unexpectedly fast rate[169], frequently exceeding the predictions of AI experts based on widely recognised benchmarks. From generating hyper-realistic images to surpassing human performance in benchmarks like handwriting recognition and grade-school maths, the trajectory of progress has been steep.
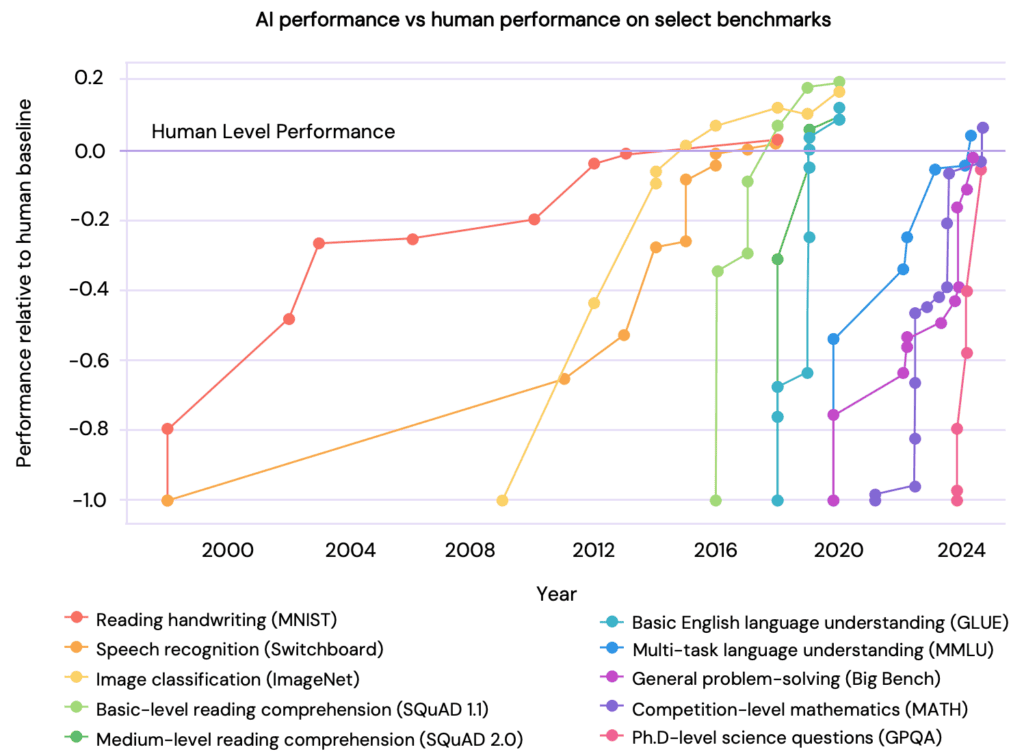
Can the current trajectory hold into the future?
Recent advancements in general-purpose AI have progressed at an unexpectedly fast rate[171], frequently exceeding the predictions of AI experts based on widely recognised benchmarks. From generating hyper-realistic images to surpassing human performance in benchmarks like handwriting recognition and grade-school maths, the trajectory of progress has been steep and transformative.
The progress we’ve observed in recent years has been largely driven by the so-called “scaling laws.”[172] Put simply, these laws describe how increasing training data, building larger models, and using more computational resources tend to result in better model performance and expanded capabilities. This trend and its technical underpinnings were the focus of our earlier piece on the state of play in advanced AI. This progress has been possible because we’ve been able—and willing—to invest the significant resources required to unlock these advancements.
But can we assume this pattern will hold in the future? Could we face limitations in providing the inputs scaling laws demand, such as sufficient data, electricity, or advanced hardware? Alternatively, might the scaling laws themselves reach a breaking point, offering diminishing returns where ever-increasing investments yield only marginal gains in AI performance?
Let’s examine both of these questions in turn.
Can we make the required inputs of data, electricity, and hardware available?
To assess whether AI can sustain its transformative trajectory, we need to examine the availability and scalability of its key inputs: data, electricity, specialised hardware, and investment. Let’s take a closer look at each. As there is a very limited amount of research being done on this, we mostly rely on the extensive modeling and subsequent report of Epoch AI in this section[173]. Further data is sorely needed in this area.
Data
Data remains a pivotal driver of AI’s growth[174]. While certain high-quality datasets could eventually become harder to source, the sheer volume of online content is still expanding, and new data sources—ranging from additional modalities to synthetic data—are emerging. These trends suggest that data constraints may not pose a significant bottleneck in the near future, allowing AI training to continue scaling at least through the rest of the decade (see Figure 11).
Electricity
Powering ever-larger AI models for training and for their use will require significantly more electricity. However, ongoing expansion in data centre capacity and improvements in energy infrastructure suggest there is enough headroom to accommodate AI’s growing power demands through the rest of the decade[175]. While energy efficiency remains an important challenge, current trends imply that power constraints may not seriously impede AI scaling in the next few years.
Specialised hardware
Specialised chips are the backbone of AI’s remarkable progress. While soaring demand can create short-term manufacturing bottlenecks, industry projections suggest a promising outlook. EpochAI’s analysis of semiconductor industry trends, including packaging capacity growth, wafer production, and fab investments, indicates that hardware supply is likely to keep pace with AI’s rising computational needs[176].
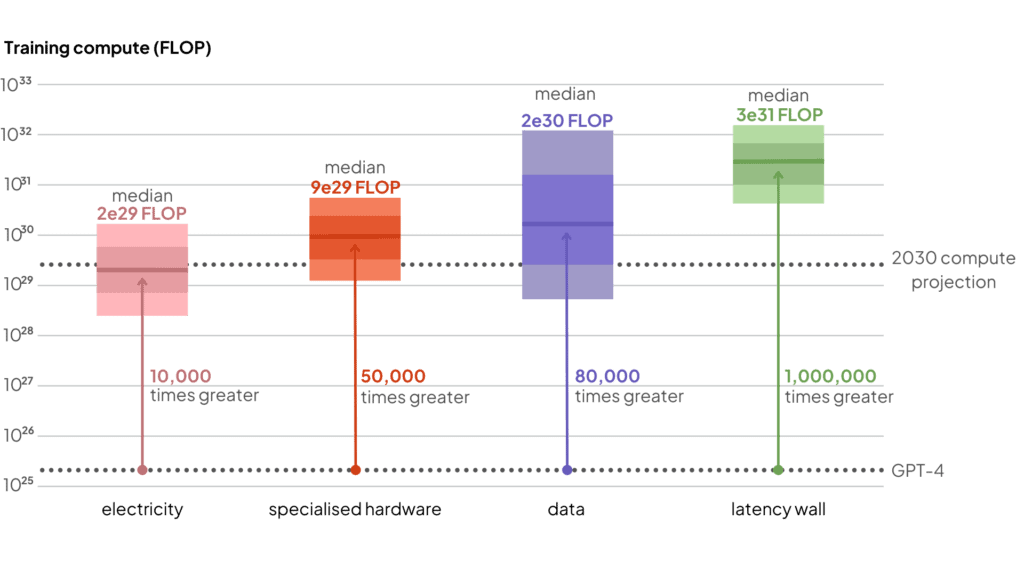
Will scaling laws continue to deliver?
While it appears likely that we can sustain the necessary inputs—data, electricity, hardware, and investment—to fuel AI’s progress, the question remains: can we count on scaling laws to continue holding?
Historically, most progress was driven by pre-training[178]: scaling up data, models, and compute to improve performance. Pre-training established the foundational capabilities, while post-training only steered those capabilities to meet specific objectives, such as generating helpful and accurate outputs.
Recent discussions and indications[179] suggest that capability gains from pre-training may be slowing[180], prompting some AI experts to speculate that deep learning might be hitting a wall[181]. If scaling laws were to break down, we could see progress stall or even require an entirely new paradigm to push AI capabilities further. In this scenario, many current expectations for transformative AI advancements could be overly optimistic.
However, the way AI capabilities are developed is evolving. While gains from pre-training may indeed be slowing, capability development is no longer confined to this single stage. Instead, advancements now occur across three distinct stages:
- Pre-Training: Scaling data and compute to establish foundational capabilities.
- Post-Training: Introducing techniques that not only steer existing capabilities but actively enhance[182] them (e.g., leveraging small, specialised datasets to improve reasoning skills).
- Runtime Optimisation: Implementing methods that improve output quality during deployment, such as enabling models to spend more time deliberating[183] before producing a response (as exemplified by OpenAI’s o1 and o3).
This multi-stage approach diversifies how capabilities are gained, reducing reliance on pre-training alone. Even as returns from pre-training diminish, innovations in post-training and runtime techniques continue to drive improvements, ensuring that progress doesn’t grind to a halt. OpenAI’s o1 and o3 models illustrate this shift vividly. The new o3 model achieved a 25% score on Epoch AI’s FrontierMath benchmark[184], a suite of problems that typically take expert mathematicians hours or even days to solve[185]. Previous AI models only managed 2% accuracy on the same benchmark, so o3’s performance is a clear leap forward. Such results suggest that rather than AI generally hitting a fundamental limit, AI development is following a a series of success S-curves (see Figure 12) with the new training methods potentially signifying beginning of the new curve.
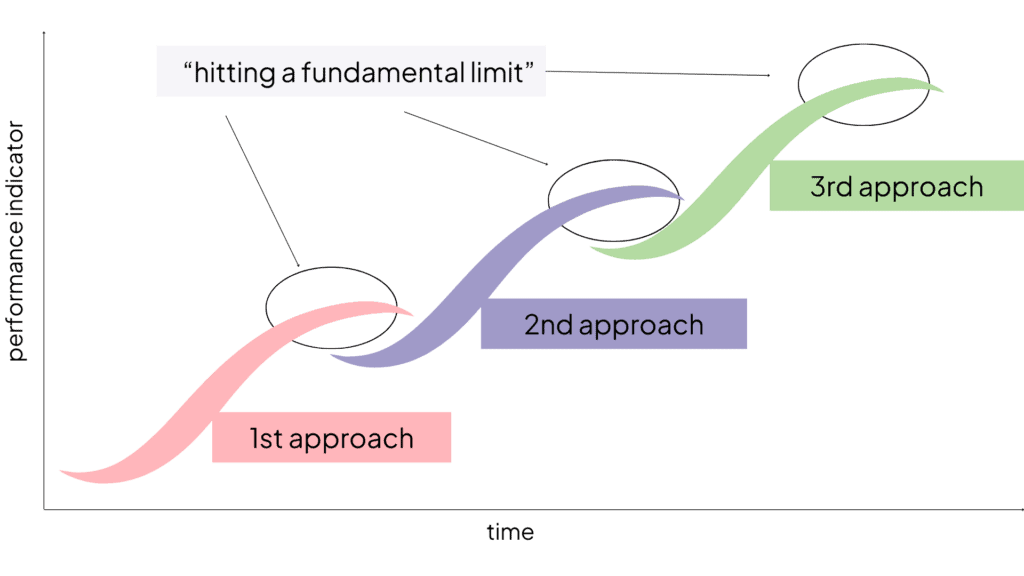
Is AI’s lack of reliability a roadblock to transformation?
One of the most significant challenges for AI systems today is reliability. Models can sometimes produce “hallucinations”—inaccurate or entirely fabricated responses—which erode user trust and hinder broader adoption. Moreover, lack of reliability might render AI models unsuitable for some high-stakes applications—such as healthcare, transportation safety, critical infrastructure management, and legal decision-making—where errors could lead to loss of life, significant harm, or systemic failure, limiting the extent to which AI can deliver some forms of transformative impact.
Reliability metrics show steady improvement, as AI developers understand this is a potential blocker. On Vectara’s Hughes Hallucination Leaderboard[187] where AI models are given a simple goal of summarising a provided document, OpenAI’s GPT-3.5 had a hallucination rate of 3.5% in November 2023, but as of January 2025, the firm’s later model GPT-4 scored 1.8% and latest o3-mini-high-reasoning model just 0.8%. However, broader evaluations in more open-ended scenarios do not always show a clear downward trend. OpenAI reports that while o1 outperformed GPT-4 on its internal hallucination benchmarks, testers observed that it sometimes generated more hallucinations[188]—particularly elaborate but incorrect responses that appeared more convincing. On TruthfulQA, a benchmark measuring model truthfulness, performance of the best AI model has improved from 58% accuracy in 2022[189] when the benchmark was published to 91.1% as of January 2025[190], which is close to the human performance of 94%.
While reliability concerns initially appear to be a major obstacle to AI adoption and might continue to be a persistent problem in high-stakes contexts, real-world evidence suggests that it is not a strong barrier for adoption and creation of some forms of unprecedented impact (as summarised earlier in Chapter 3). Companies are already finding valuable applications for AI despite its imperfections, and the technology continues to improve rapidly. Rather than waiting for perfect reliability, organisations are learning to leverage AI’s strengths. Further, some paths to transformative impact—like accelerating scientific progress—don’t require widespread adoption or balanced human-like skillset and might not require perfect reliability either.
When do experts anticipate AI to become transformative?
The trajectory of artificial intelligence is inherently uncertain, and expert predictions in this and many other fields have historically shown significant limitations. Nevertheless, perspectives from industry leaders, researchers, and forecasters can provide valuable insights into its potential and the challenges ahead. These experts offer unique vantage points on AI’s development, though like any forward-looking assessments, they represent possibilities rather than certainties. By examining their views, we’re better placed to assess whether the prospect of transformative AI in the near term is something we ought to take seriously—and what might make it more or less likely. While they can’t tell us when exactly AI will become transformative, these views help clarify whether the possibility deserves attention.
Industry insiders: Balancing insight and bias
Professionals working within AI labs and companies have unparalleled insight into the technology’s capabilities and development pipelines. They are the only ones having access to the “future” of the technology: seeing first glimpses of new capabilities and experimenting with them before they are broadly deployed to the public. However, their perspectives may be shaped by incentives to emphasise the promise of their work, which can attract investments or reinforce the importance of their field. Historically[191], AI experts often predicted major advancements 15 to 25 years into the future, a timeline that conveniently avoids immediate scrutiny.
In recent years, predictions have shifted significantly closer, reflecting growing confidence among those closest to the technology. For example, OpenAI’s CEO Sam Altman has expressed[192] confidence that “In 2025, we may see the first AI agents ‘join the workforce’ and materially change the output of companies” and that “AGI will probably get developed during [Trump’s] term.” Similarly, Dario Amodei, CEO of Anthropic, has suggested[193] that AGI could arrive as soon as 2026 or 2027 unless something goes wrong. In fact, he has stated[194] that what he has seen within Anthropic in recent months has led him to believe that AI will surpass almost all humans at almost all tasks within two to three years. These projections suggest that industry insiders are confident enough in the trajectory of current advancements to risk their reputations on bold, near-term forecasts, although making bold claims might also serve them as a way to attract investors.
McKinsey—not itself an AI company, but instead known for its pragmatic and market-focused analysis—highlights the transformative potential of AI, stating that[195] “increasing investments in these tools could result in agentic systems achieving notable milestones and being deployed at scale over the next few years”. Their focus on agent-driven automation emphasises its potential to revolutionise industries by increasing speed and efficiency in workflows. While they caution that agent technology is still in its infancy, they point to growing investments and the likelihood of achieving large-scale deployment soon. Importantly, McKinsey’s perspective has evolved, with their latest adoption scenarios accounting for generative AI developments. They now (as of their July 2024 report[196]) predict that 50% of time spent on 2023 work activities could be automated between 2030 and 2060, with a midpoint of 2045, which is roughly a decade earlier[197] than their previous 2016 estimate (see Figure 13). This adjustment signals the accelerated pace of progress and its potential to reshape industries worldwide.
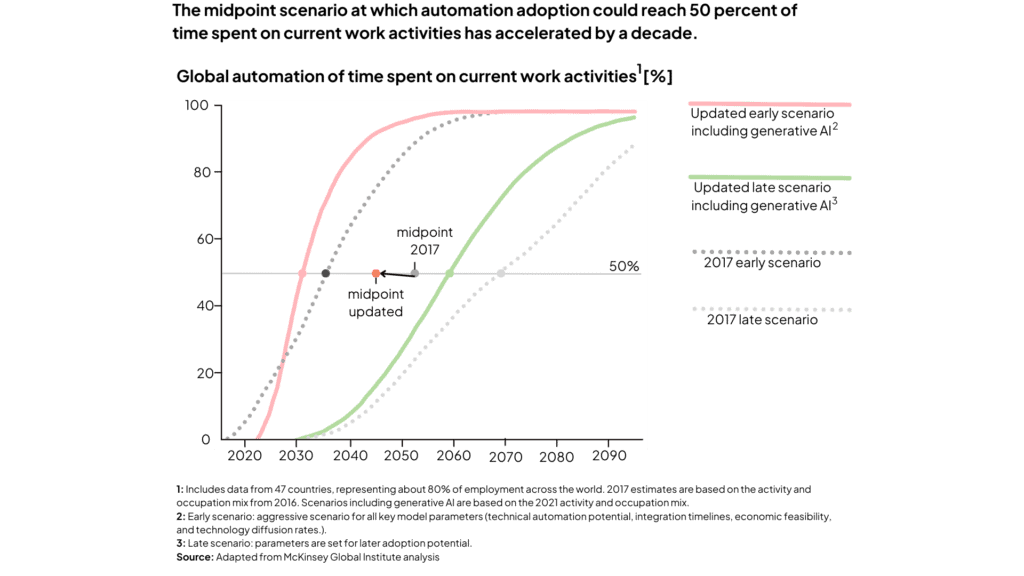
Academia & researchers
Academic researchers provide a critical, often independent perspective on AI’s progress, leveraging their empirical work and deep theoretical expertise. Their insights are less prone to be influenced by market pressures, offering a valuable counterbalance to industry narratives.
Recent surveys show that academic forecasts for achieving “human-level” AI have accelerated significantly. For instance, examining the Expert Surveys on Progress in AI[199], a comparison between 2016 and 2022 surveys conducted with 2,778 researchers, shows that predictions shifted by only about one year. However, the difference between the 2022 and 2023 surveys reveals a dramatic surge in expectations, with predictions for reaching transformative milestones moving forward by one to five decades in just a single year. This shift reflects the researchers’ increasing confidence in the rapid pace of advancements in the field.
Crowd forecasts
Crowd forecasts, such as prediction markets and forecasting tournaments, aggregate diverse perspectives, often combining insights from domain experts and informed generalists, and often use financial incentives to reward accuracy. Studies[200] show[201] that[202] prediction markets and crowd forecasting platforms are among the most reliable methods for aggregating probabilistic judgments, consistently outperforming individual experts and often matching or surpassing expert panels. This makes them a valuable tool for gauging collective confidence in AI’s trajectory.
Trends from crowd forecasts[203] align with other expert analyses, showing heightened optimism for near-term advancements. For example, forecasts[204] for achieving weakly general AI —an AI system capable of performing a wide range of tasks at a level comparable to a typical college-educated human, including passing tests like the Turing Test and SATs—have shifted dramatically, moving from the distant 2040s to centring around 2027. Similarly, forecasts[205] on when to expect the first general AI system—which they define as a system that can pass a rigorous adversarial Turing test, demonstrate advanced robotics capabilities, and achieve expert-level accuracy on a range of intellectual and technical tasks—have moved from around 2050 to 2031.
This trend is also evident in predictions about more direct applications of AI: the likelihood that AI will be able to generate 10,000 lines of bug-free code before 2030 increased from 50% in 2022 to over 90% in 2024[206]. Shifts like these suggest a growing belief that transformative capabilities may emerge much sooner than previously expected.
Summary: The convergence of accelerating capabilities, expert forecasts, and industry investments suggests transformative AI could emerge in years rather than decades. Progress has been remarkably rapid, with AI systems mastering capabilities much faster than experts anticipated, while former bottlenecks like compute, data, and reliability are being systematically addressed through both technical innovation and massive investment. Most tellingly, timelines from diverse sources—industry leaders, academic researchers, and prediction markets—have collectively shifted forward by decades in just the past year, with many now suggesting transformative capabilities could emerge in the next few years. As we argue in the Conclusion, this might make current policy and governance decisions disproportionately consequential. |
Conclusion
Over the course of this report, we have seen a tangled mix of promise and concern woven into the AI landscape. On the one hand, there is clear momentum: AI’s capabilities have grown quickly, attracting unprecedented levels of investment, adoption, and genuine achievements. From breakthroughs in protein folding and advanced mathematics to tangible boosts in workplace productivity, AI is proving that its core technologies can drive meaningful change. The steadily falling costs of training and deploying AI systems, alongside the ever-broadening range of tasks they can handle, suggest that these developments will continue to spread—potentially revolutionising industries at a rate that is hard to imagine.
Yet every step forward brings its own uncertainties. Some AI applications are still fragile, failing at tasks that humans find trivial. Companies may be throwing money at AI as part of a bandwagon effect, and performance on benchmarks does not always translate cleanly into real-world reliability. Nevertheless, taken as a whole, the evidence suggests that AI holds the potential to drive transformative changes in the coming years. While some pockets of overblown claims exist, it would be a mistake to confidently dismiss AI as “overhyped” in general.
The timeline for so-called transformative impacts could be shorter than many had assumed—years rather than decades—particularly as autonomous, “agentic” systems become ever more integrated into practical workflows. However, this projected path depends on critical factors like data availability, power supply, hardware innovation, and the continuing willingness of companies and governments to fund large-scale development. That being said, the most extensive analysis of these factors that we could find indicates that all these factors likely will stay sufficiently available at least until 2030.
The convergence of expert opinions, technological trajectories, and market signals suggests that significant disruption could begin within the next few years, not decades. |
For policymakers and stakeholders, this creates a challenging environment. AI could bring transformative changes to society—however, because it may be developed quickly, we likely have only a short window of opportunity to guide its development. In any case, the decisions we make in the next few years are likely to have a disproportionate impact on how AI reshapes society.
As we navigate this critical phase, staying level-headed is key. The combination of uncertainty and potential stakes calls for more foresight—including monitoring, early warning signals, and ongoing assessment—to adapt as AI evolves.
In the face of this uncertainty, policy needs to be robust to a range of possibilities, and in particular, we need to prepare contingency plans for the scenarios where we see the most transformative changes to society. Because AI could become truly transformative, we need to think bigger from a societal perspective: what are the incentives that shape the development of AI, what are the consequences of these incentives in terms of what risks and benefits AI systems will bring about, who controls them, how they are governed, and how are their risks and benefits distributed. These are questions that will all matter greatly for EU sovereignty and societal wellbeing.
Lastly, it is worth noting that we still do not have science of safe AI[207]. The EU cannot gamble on foreign, profit-driven companies to solve such a high-stakes challenge, nor can it rely on regulation alone. Instead, the EU should invest heavily in trustworthy AI, combining balanced oversight, and building the state capacity to understand and govern these technologies for the benefit of both present and future generations. The future of AI is neither fixed nor beyond our influence—we have agency and the time to shape our path is now.
Acknowledgements
We would like to express our sincere gratitude to Aaron Maniam, David Timis, Kristina Fort, Leonardo Quattrucci, Pawel Swieboda, and many of our CFG colleagues for their valuable feedback and insights on this report. Their thoughtful comments and suggestions have significantly improved the quality of our work. Any remaining errors or omissions are our own.
Endnotes
[1] Janků, D., Reddel, F., Graabak, J. and Reddel, M., ‘Beyond the AI Hype – The FAQ: Common Questions Around AI’s Transformative Potential’, Centre for Future Generations, 2025, available at: https://cfg.eu/beyond-the-ai-hype-faq/
[2] Markelius, A., Wright, C., Kuiper, J., Delille, N. and Kuo, Y., ‘The Mechanisms of AI Hype and Its Planetary and Social Costs’, AI and Ethics, vol. 4, 2024, available at: https://link.springer.com/article/10.1007/s43681-024-00461-2.
[3] Thais, S., ‘Misrepresented Technological Solutions in Imagined Futures: The Origins and Dangers of AI Hype in the Research Community’, Proceedings of the AAAI/ACM Conference on AI, Ethics, and Society, vol. 7, 2024, pp. 1455-1465, available at https://ojs.aaai.org/index.php/AIES/article/view/31737/33904.
[4] Powers, D., ‘Notes on Hype’, International Journal of Communication, vol. 6, 2012, p. 17, available at https://ijoc.org/index.php/ijoc/article/view/1441/736.
[5] West, D.M. and Allen, J.R., ‘How Artificial Intelligence is Transforming the World’, Brookings, 2018, available at https://www.brookings.edu/articles/how-artificial-intelligence-is-transforming-the-world/.
[6] Karnofsky, H., ‘Some Background on Our Views Regarding Advanced Artificial Intelligence’, Open Philanthropy, 2016, available at https://www.openphilanthropy.org/research/some-background-on-our-views-regarding-advanced-artificial-intelligence/.
[7] Allan Dafoe, ‘AI Governance: A Research Agenda’, Centre for the Governance of AI, Future of Humanity Institute, University of Oxford, 2018, https://cdn.governance.ai/GovAI-Research-Agenda.pdf
[8] Gruetzemacher, R. and Whittlestone, J., ‘Defining and Unpacking Transformative AI’, arXiv, 2019, available at https://arxiv.org/vc/arxiv/papers/1912/00747v1.pdf.
[9] Karnofsky, H., ‘Some Background on Our Views Regarding Advanced Artificial Intelligence’, Open Philanthropy, 2016, available at https://www.openphilanthropy.org/research/some-background-on-our-views-regarding-advanced-artificial-intelligence/.
[10] Okemwa, A., ‘A Leaked Document Suggests OpenAI Will Hit AGI When It Builds an AI System That Can Generate up to $100 Billion in Profit — but the ChatGPT Maker Could Endure a Massive $44 Billion Loss Before Seeing Profit in 2029 Partly Due to Microsoft Tie-up’, Windows Central, 2025, available at https://www.windowscentral.com/software-apps/a-leaked-document-suggests-openai-will-hit-agi-when-it-builds-an-ai-system-that-can-generate-up-to-usd100-billion-in-profit-but-the-chatgpt-maker-could-endure-a-massive-usd44-billion-loss-before-seeing-profit-in-2029-partly-due-to-microsoft-tie-up.
[11] Dafoe, A., ‘AI Governance: A Research Agenda’, Centre for the Governance of AI, Future of Humanity Institute, University of Oxford, 2018, available at https://cdn.governance.ai/GovAI-Research-Agenda.pdf.
[12] Stanford HAI, ‘HAI AI‑Index‑Report‑2024’, Stanford HAI, 2024. Available at: https://aiindex.stanford.edu/wp-content/uploads/2024/05/HAI_AI-Index-Report-2024.pdf
[13] Janků, D., Reddel, F., Graabak, J. and Reddel, M., ‘Beyond the AI Hype – The FAQ: Common Questions Around AI’s Transformative Potential’, Centre for Future Generations, 2025, available at: https://cfg.eu/beyond-the-ai-hype-faq/
[14] Google Trends. ‘AI’, Google Trends, 2025. Available at: https://trends.google.com/trends/explore?date=today%205-y&q=AI&hl=en
[15] Ittefaq, M. et al., ‘Global News Media Coverage of Artificial Intelligence (AI): A Comparative Analysis’, ScienceDirect, 2025, available at https://www.sciencedirect.com/science/article/abs/pii/S0736585324001278.
[16] Xian, L. et al., ‘Landscape of Generative AI in Global News: Topics, Sentiments, and Spatiotemporal Analysis’, ArXiv, 2024, available at https://arxiv.org/pdf/2401.08899.
[17] Stanford HAI, ‘HAI AI‑Index‑Report‑2024’, Stanford HAI, 2024. Available at: https://aiindex.stanford.edu/wp-content/uploads/2024/05/HAI_AI-Index-Report-2024.pdf
[18] Ipsos, ‘Ipsos-AI-Monitor-2024-Final-APAC’, Ipsos, 2024, available at https://www.ipsos.com/sites/default/files/ct/news/documents/2024-06/Ipsos-AI-Monitor-2024-final-APAC.pdf.
[19] Backlinko, ‘ChatGPT Statistics 2025: How Many People Use ChatGPT?’, Backlinko, 2025, available at https://backlinko.com/chatgpt-stats.
[20] Reuters, ‘400 Million Weekly Users’, Reuters, 2025, available at https://www.reuters.com/technology/artificial-intelligence/openais-weekly-active-users-surpass-400-million-2025-02-20/
[21] Pew Research Center, ‘Americans Increasingly Using ChatGPT, but Few Trust Its 2024 Election Information’, Pew Research Center, 2024, available at https://www.pewresearch.org/short-reads/2024/03/26/americans-use-of-chatgpt-is-ticking-up-but-few-trust-its-election-information/.
[22] Ipsos, ‘Ipsos-AI-Monitor-2024-Final-APAC’, Ipsos, 2024, available at https://www.ipsos.com/sites/default/files/ct/news/documents/2024-06/Ipsos-AI-Monitor-2024-final-APAC.pdf.
[23] Ipsos, ‘Ipsos Global AI 2023 Report’, Ipsos, 2023, available at https://www.ipsos.com/sites/default/files/ct/news/documents/2023-07/Ipsos%20Global%20AI%202023%20Report-WEB_0.pdf.
[24] Ipsos, ‘Ipsos-AI-Monitor-2024-Final-APAC’, Ipsos, 2024, available at https://www.ipsos.com/sites/default/files/ct/news/documents/2024-06/Ipsos-AI-Monitor-2024-final-APAC.pdf.
[25] Stanford HAI, ‘HAI AI‑Index‑Report‑2024’, Stanford HAI, 2024. Available at: https://aiindex.stanford.edu/wp-content/uploads/2024/05/HAI_AI-Index-Report-2024.pdf
[26] Stanford HAI, ‘HAI AI‑Index‑Report‑2024’, Stanford HAI, 2024. Available at: https://aiindex.stanford.edu/wp-content/uploads/2024/05/HAI_AI-Index-Report-2024.pdf
[27] Stanford HAI, ‘HAI AI‑Index‑Report‑2024’, Stanford HAI, 2024. Available at: https://aiindex.stanford.edu/wp-content/uploads/2024/05/HAI_AI-Index-Report-2024.pdf
[28] Kindig, B., ‘AI Spending to Exceed a Quarter Trillion Next Year’, Forbes, 2024, available at https://www.forbes.com/sites/bethkindig/2024/11/14/ai-spending-to-exceed-a-quarter-trillion-next-year/.
[29] Financial Times, ‘Google DeepMind Duo Share Nobel Chemistry Prize with US Biochemist’, Financial Times, 2024, available at https://www.ft.com/content/4541c07b-f5d8-40bd-b83c-12c0fd662bd9.
[30] Parasnis, S., ‘Bank of China announces 1 Trillion Yuan AI Industry investment’, Medianama, 2025. Available at: https://www.medianama.com/2025/01/223-bank-of-china-announces-1-trillion-yuan-ai-industry-investment/
[31] Wold, J.W., ‘Von der Leyen Launches World’s Largest Public‑Private Partnership To Win AI Race’, EURACTIV, 2025. Available at: https://www.euractiv.com/section/tech/news/von-der-leyen-launches-worlds-largest-public-private-partnership-to-win-ai-race/
[32] Seeking Alpha, ‘Microsoft Corporation (MSFT) Q1 2025 Earnings Call Transcript’, Seeking Alpha, 2025, available at https://seekingalpha.com/article/4731223-microsoft-corporation-msft-q1-2025-earnings-call-transcript.
[33] Kindig, B., ‘Microsoft – AI Will Help Drive $100 Billion in Revenue by 2027’, IO-fund.com, 2023, available at https://io-fund.com/cloud-software/software/microsoft-ai-will-help-drive-100-billion-in-revenue.
[34] Kindig, B., ‘AI Spending to Exceed a Quarter Trillion Next Year’, Forbes, 2024, available at https://www.forbes.com/sites/bethkindig/2024/11/14/ai-spending-to-exceed-a-quarter-trillion-next-year/.
[35] Buchholz, K., ‘Chart: The Extreme Cost of Training AI Models’, Statista, 2024, available at https://www.statista.com/chart/33114/estimated-cost-of-training-selected-ai-models/.
[36] Knight, W., ‘OpenAI’s CEO Says the Age of Giant AI Models Is Already Over’, WIRED, 2023, available at https://web.archive.org/web/20230418190335/https://www.wired.com/story/openai-ceo-sam-altman-the-age-of-giant-ai-models-is-already-over/.
[37] Heeter, M., Efrati, A. and Palazzolo, S., ‘OpenAI’s Annualized Revenue Tops $1.6 Billion as Customers Shrug Off CEO Drama’, The Information, available at https://www.theinformation.com/articles/openais-annualized-revenue-tops-1-6-billion-as-customers-shrug-off-ceo-drama.
[38] Hu, K. and Cai, K., ‘OpenAI is Predicting Its Revenue… Speaking on Condition of Anonymity’, Reuters, 2024, available at https://www.reuters.com/technology/artificial-intelligence/openai-sees-116-billion-revenue-next-year-offers-thrive-chance-invest-again-2025-2024-09-28/#:~:text=OpenAI%20is%20predicting%20its%20revenue,speaking%20on%20condition%20of%20anonymity.
[39] ibid.
[40] PYMNTS, ‘OpenAI Plans Grand Expansion While Losing Billions. Sound Familiar?’, PYMNTS, 2025, available at https://www.pymnts.com/artificial-intelligence-2/2025/openai-plans-grand-expansion-while-losing-billions-will-it-survive/#:~:text=It%20has%20raised%20%2421.9%20billion,by%20The%20Information%20last%20October
[41] Tsarathustra, ‘Dane Vahey of OpenAI Says the Cost…’, X, 2024, available at https://x.com/tsarnick/status/1838401544557072751.
[42] Wikipedia, ‘Solar Cell’, Wikipedia, available at https://en.wikipedia.org/wiki/Solar_cell.
[43] Our World in Data, ‘Solar PV Prices’, Our World in Data, 2024, available at https://ourworldindata.org/grapher/solar-pv-prices.
[44] Our World in Data, ‘Cost of Sequencing a Full Human Genome’, Our World in Data, 2023, available at https://ourworldindata.org/grapher/cost-of-sequencing-a-full-human-genome.
[45] Tsarathustra, ‘Dane Vahey of OpenAI Says the Cost…’, X, 2024, available at https://x.com/tsarnick/status/1838401544557072751.
[46] OpenAI, ‘Pricing’, OpenAI, 2023, available at https://web.archive.org/web/20230401144811/https://openai.com/pricing.
[47] Exponential View, ‘From ChatGPT to a Billion Agents’, Exponential View, 2024, available at https://www.exponentialview.co/p/from-chatgpt-to-a-billion-agents.
[48] AI Snake Oil, ‘GPT-4 and Professional Benchmarks: The Wrong Answer to the Wrong Question’, AI Snake Oil, 2023, available at https://www.aisnakeoil.com/p/gpt-4-and-professional-benchmarks.
[49] Eriksson, M. et al., ‘Can We Trust AI Benchmarks? An Interdisciplinary Review of Current Issues in AI Evaluation’, ArXiv, 2025, available at https://arxiv.org/abs/2502.06559.
[50] OpenAI Developer Community, ‘Incorrect Count of ‘r’ Characters in the Word ‘strawberry’ – Use Cases and Examples’, OpenAI Developer Community, 2024, available at https://community.openai.com/t/incorrect-count-of-r-characters-in-the-word-strawberry/829618.
[51] Newton, C., ‘Google’s AI Search Setback’, Platformer, 2024, available at https://www.platformer.news/google-ai-overviews-eat-rocks-glue-pizza/.
[52] Chervonyi, Y. et al., ‘Gold-Medalist Performance in Solving Olympiad Geometry with AlphaGeometry2’, arXiv, 2025, available at https://arxiv.org/pdf/2502.03544.
[53] Ars Technica, ‘OpenAI Announces o3 and o3-mini, Its Next Simulated Reasoning Models’, Ars Technica, 2024, available at https://arstechnica.com/information-technology/2024/12/openai-announces-o3-and-o3-mini-its-next-simulated-reasoning-models/.
[54] Epoch AI, ‘FrontierMath: LLM Benchmark For Advanced AI Math Reasoning’, Epoch AI, 2024. Available at: https://epoch.ai/frontiermath
[55] Google DeepMind, ‘AlphaFold: A Solution to a 50-Year-Old Grand Challenge in Biology’, Google DeepMind, 2020, available at https://deepmind.google/discover/blog/alphafold-a-solution-to-a-50-year-old-grand-challenge-in-biology/.
[56] Financial Times, ‘Google DeepMind Duo Share Nobel Chemistry Prize with US Biochemist’, Financial Times, 2024, available at https://www.ft.com/content/4541c07b-f5d8-40bd-b83c-12c0fd662bd9.
[57] Ars Technica, ‘OpenAI Announces o3 and o3-mini, Its Next Simulated Reasoning Models’, Ars Technica, 2024, available at https://arstechnica.com/information-technology/2024/12/openai-announces-o3-and-o3-mini-its-next-simulated-reasoning-models/.
[58] Lin, S., Hilton, J., and Evans, O., ‘TruthfulQA: Measuring How Models Mimic Human Falsehoods’, arXiv, 2021, https://arxiv.org/abs/2109.07958
[59] Nobel Prize, ‘The Nobel Prize in Physics 2024 Press Release’, Nobel Prize, 2024, available at https://www.nobelprize.org/prizes/physics/2024/press-release/.
[60] The Nobel Prize, ‘The Nobel Prize in Chemistry 2024 Press Release’, Nobel Prize, 2024, available at https://www.nobelprize.org/prizes/chemistry/2024/press-release/.
[61] Benaich, N. and Chalmers, A., ‘AI Isn’t The Dotcom Bubble’, AIR STREET PRESS, 2024. Available at: https://press.airstreet.com/p/ai-isnt-the-dotcom-bubble
[62] Goh, E. et al., ‘Large Language Model Influence on Diagnostic Reasoning: A Randomized Clinical Trial’, JAMA Network Open, 2024, available at https://jamanetwork.com/journals/jamanetworkopen/fullarticle/2825395.
[63] Rutledge, G.W., ‘Diagnostic Accuracy of GPT-4 on Common Clinical Scenarios and Challenging Cases’, PMC, available at https://pmc.ncbi.nlm.nih.gov/articles/PMC11257049/.
[64] McDuff, D. et al., ‘Towards Accurate Differential Diagnosis with Large Language Models’, ArXiv, 2023, available at https://arxiv.org/abs/2312.00164.
[65] Rao, A. et al., ‘Assessing the Utility of ChatGPT Throughout the Entire Clinical Workflow: Development and Usability Study’, Journal of Medical Internet Research, 2023, available at https://www.jmir.org/2023/1/e48659/.
[66] Hernström, V. et al., ‘Screening Performance and Characteristics of Breast Cancer Detected in the Mammography Screening with Artificial Intelligence Trial (MASAI): A Randomised, Controlled, Parallel-Group, Non-Inferiority, Single-Blinded, Screening Accuracy Study’, The Lancet, 2025, available at https://www.thelancet.com/journals/landig/article/PIIS2589-7500%2824%2900267-X/fulltext.
[67] Wan, F. et al., ‘Machine Learning for Antimicrobial Peptide Identification and Design’, Nature Reviews Bioengineering, 2024, available at https://www.nature.com/articles/s44222-024-00152-x.
[68] Penn Bioengineering Blog, ‘Artificial Intelligence to Accelerate Antibiotic Discovery’, Penn Bioengineering Blog, 2024, available at https://beblog.seas.upenn.edu/artificial-intelligence-to-accelerate-antibiotic-discovery/.
[69] Talat, A. and Khan, A., ‘Artificial Intelligence as a Smart Approach to Develop Antimicrobial Drug Molecules: A Paradigm to Combat Drug-Resistant Infections’, ScienceDirect, 2023, available at https://www.sciencedirect.com/science/article/abs/pii/S1359644623000077?via%3Dihub.
[70] Krishnan, N., ‘OpenAI’s O3: A New Frontier in AI Reasoning Models’, Towards AI, 2024, https://pub.towardsai.net/openais-o3-a-new-frontier-in-ai-reasoning-models-a02999246ffe
[71] Lu, Z. et al., ‘Machine Learning Driven Design of High-Performance Al Alloys’, OAEPublish, 2024, available at https://www.oaepublish.com/articles/jmi.2024.21.
[72] Merchant, A. et al., ‘Scaling Deep Learning for Materials Discovery’, Nature, 2023, available at https://www.nature.com/articles/s41586-023-06735-9.
[73] Gurnani, R. et al., ‘AI-assisted discovery of high-temperature dielectrics for energy storage’, Nature, 2024, https://www.nature.com/articles/s41467-024-50413-x.pdf
[74]ARC Prize, ‘ARC Prize – What Is ARC-AGI?’, ARC Prize, available at https://arcprize.org/arc.
[75] For example, when shown how a simple game works where red pieces can ‘eat’ blue pieces but only if they’re smaller, the AI can figure out what would happen in complex new situations with different sizes and arrangements, showing it truly understands the rules rather than just memorising outcomes.
[76] OpenAI o3 Breakthrough High Score On ARC-AGI-pub’, ARC Prize, 2024, https://arcprize.org/blog/oai-o3-pub-breakthrough
[77] LeGris, S., Vong, W. K., Lake, B. M., and Gureckis, T. M., ‘H-ARC: A Robust Estimate of Human Performance on the Abstraction and Reasoning Corpus Benchmark’, arXiv, 2024, https://arxiv.org/pdf/2409.01374
[78] TIME, ‘Francois Chollet: The 100 Most Influential People in AI 2024’, TIME, 2024, available at https://time.com/7012823/francois-chollet/.
[79] MMMU Benchmark, ‘MMMU’, MMMU Benchmark, available at https://mmmu-benchmark.github.io/#leaderboard.
[80] Creamer, E., ‘AI Poetry Rated Better Than Poems Written by Humans’, The Guardian, 2024, available at https://www.theguardian.com/books/2024/nov/18/ai-poetry-rated-better-than-poems-written-by-humans-study-shows?ref=platformer.news.
[81] Lin, S., Hilton, J., and Evans, O., ‘TruthfulQA: Measuring How Models Mimic Human Falsehoods’, arXiv, 2021, https://arxiv.org/abs/2109.07958
[82] Simpson, W., ‘The First Ever AI-Generated Track to Hit the Charts Has Landed in Germany, and People Aren’t Happy’, MusicRadar, 2024, available at https://www.musicradar.com/news/first-ever-ai-chart-germany.
[83] Ding, J., Technology and the Rise of Great Powers: How Diffusion Shapes Economic Competition, Princeton, NJ, Princeton University Press, 2024, available at https://press.princeton.edu/books/paperback/9780691260341/technology-and-the-rise-of-great-powers?srsltid=AfmBOopELBKgsfq0tTJY0zxeFzxfatALnpKgDfT-I5q4MllVIWaLnGjl.
[84] Eurostat, ‘Use of Artificial Intelligence in Enterprises’, Eurostat, 2025, available at https://ec.europa.eu/eurostat/statistics-explained/index.php?title=Use_of_artificial_intelligence_in_enterprises#:~:text=23%20January%202025-,Highlights,the%20information%20and%20communication%20sector.
[85] Eurostat, ‘Use of Artificial Intelligence in Enterprises’, Eurostat, 2025, available at https://ec.europa.eu/eurostat/statistics-explained/index.php?title=Use_of_artificial_intelligence_in_enterprises#:~:text=23%20January%202025-,Highlights,the%20information%20and%20communication%20sector.
[86] Strand Partners, ‘The Digital Decade Vision’, Unlocking Europe’s AI Potential, available at https://www.unlockingeuropesaipotential.com/executivesummary.
[87] Deloitte Insights, ‘Europeans Are Optimistic About Generative AI But There Is More To Do To Close The Trust Gap’, Deloitte Insights, 2024, available at https://www2.deloitte.com/us/en/insights/topics/digital-transformation/trust-in-generative-ai-in-europe.html.
[88] McKinsey, ‘The State of AI in 2024’, McKinsey & Company, 2024, available at https://www.mckinsey.com/capabilities/quantumblack/our-insights/the-state-of-ai.
[89] McKinsey, ‘The State of AI in 2024’, McKinsey & Company, 2024, available at https://www.mckinsey.com/capabilities/quantumblack/our-insights/the-state-of-ai.
[90] McClain, C., Kennedy, B., Gottfried, J., Anderson, M., and Pasquini, G., ‘How the U.S. Public and AI Experts View Artificial Intelligence’, Pew Research Center, 2025, available at https://www.pewresearch.org/2025/04/03/artificial-intelligence-in-daily-life-views-and-experiences/.
[91] Deloitte Insights, ‘Europeans Are Optimistic About Generative AI But There Is More To Do To Close The Trust Gap’, Deloitte Insights, 2024, available at https://www2.deloitte.com/us/en/insights/topics/digital-transformation/trust-in-generative-ai-in-europe.html.
[92] Deloitte, ‘Now Decides Next: Moving From Potential To Performance’, Deloitte, 2024, available at https://www2.deloitte.com/content/dam/Deloitte/us/Documents/consulting/us-state-of-gen-ai-q3.pdf.
[93] McKinsey, ‘The State of AI in 2024’, McKinsey & Company, 2024, available at https://www.mckinsey.com/capabilities/quantumblack/our-insights/the-state-of-ai.
[94] Hu, K., ‘ChatGPT Sets Record for Fastest-Growing User Base – Analyst Note’, Reuters, 2023, available at https://www.reuters.com/technology/chatgpt-sets-record-fastest-growing-user-base-analyst-note-2023-02-01/.
[95] Reuters, ‘400 Million Weekly Users’, Reuters, 2025, available at https://www.reuters.com/technology/artificial-intelligence/openais-weekly-active-users-surpass-400-million-2025-02-20/
[96] Pew Research Center, ‘Americans Increasingly Using ChatGPT, but Few Trust Its 2024 Election Information’, Pew Research Center, 2024, available at https://www.pewresearch.org/short-reads/2024/03/26/americans-use-of-chatgpt-is-ticking-up-but-few-trust-its-election-information/.
[97] Our World in Data, ‘Share of United States Households Using Specific Technologies’, Our World in Data, n.d., available at https://ourworldindata.org/grapher/technology-adoption-by-households-in-the-united-states.
[98] Pew Research Center, ‘Americans Increasingly Using ChatGPT, but Few Trust Its 2024 Election Information’, Pew Research Center, 2024, available at https://www.pewresearch.org/short-reads/2024/03/26/americans-use-of-chatgpt-is-ticking-up-but-few-trust-its-election-information/.
[99] Bick, A., Blandin, A., and Deming, D.J., ‘The Rapid Adoption of Generative AI’, 2024, available at https://889099f7-c025-4d8a-9e78-9d2a22e8040f.usrfiles.com/ugd/889099_fa955a5012dd45abb84dd647ce02de95.pdf.
[100] YouGov, ‘Do Americans Think AI Will Have a Positive or Negative Impact on Society?’, YouGov, 2025, available at https://today.yougov.com/technology/articles/51368-do-americans-think-ai-will-have-positive-or-negative-impact-society-artificial-intelligence-poll.
[101] McKinsey, ‘The State of AI in 2024’, McKinsey & Company, 2024, available at https://www.mckinsey.com/capabilities/quantumblack/our-insights/the-state-of-ai.
[102] McKinsey, ‘The State of AI in 2024’, McKinsey & Company, 2024, available at https://www.mckinsey.com/capabilities/quantumblack/our-insights/the-state-of-ai.
[103] Google, ‘Alphabet Q3 Earnings Call: CEO Sundar Pichai’s Remarks’, Google (blog), 2024, https://blog.google/inside-google/message-ceo/alphabet-earnings-q3-2024/#full-stack-approach
[104] Jassy, A., ‘One of the Most Tedious (but Critical Tasks) for Software Development Teams…’, X, 2024, available at https://x.com/ajassy/status/1826608791741493281?lang=en.
[105] Goldman Sachs, ‘AI Is Showing ‘Very Positive’ Signs of Eventually Boosting GDP and Productivity’, Goldman Sachs, 2024, available at https://www.goldmansachs.com/insights/articles/AI-is-showing-very-positive-signs-of-boosting-gdp.
[106] Cambon, A. et al., ‘Early LLM-based Tools for Enterprise Information Workers Likely Provide Meaningful Boosts to Productivity’, Microsoft Research, 2023, available at https://www.microsoft.com/en-us/research/wp-content/uploads/2023/12/AI-and-Productivity-Report-First-Edition.pdf.
[107] Dell’Acqua, F. et al., ‘Navigating The Jagged Technological Frontier: Field Experimental Evidence Of The Effects Of AI On Knowledge Worker Productivity And Quality’, Harvard Business School, 2023, available at https://www.hbs.edu/faculty/Pages/item.aspx?num=64700.
[108] Acemoglu, D. and Restrepo, P., ‘Automation, Artificial Intelligence, and the Future of Work’, NBER Working Paper, no. 31161, 2023, available at https://www.nber.org/papers/w31161.
[109] Choi, J.H., Monahan, A. and Schwarcz, D., ‘Lawyering in the Age of Artificial Intelligence’, SSRN, 2023, available at https://papers.ssrn.com/sol3/papers.cfm?abstract_id=4626276.
[110] Toner-Rodgers, A., ‘Artificial Intelligence, Scientific Discovery, and Product Innovation’, NBER Conference Papers, 2024, available at https://conference.nber.org/conf_papers/f210475.pdf.
[111] OECD, Artificial Intelligence in Science: Challenges, Opportunities and the Future of Research, Paris, France, OECD Publishing, 2023, available at: https://www.oecd.org/content/dam/oecd/en/publications/reports/2023/06/artificial-intelligence-in-science_4f3d6efd/a8d820bd-en.pdf.
[112] Sutherland, W.J. et al., ‘Quantifying the Impact and Relevance of Scientific Research’, PLOS ONE, vol. 6, no. 11, 2011, available at https://journals.plos.org/plosone/article?id=10.1371/journal.pone.0027537.
[113] Libert, B., ‘Beyond Moonshots: AI Agents Will Come to Earth in 2025’, Forbes, 2024, available at https://www.forbes.com/sites/libertbarry/2024/11/22/beyond-moonshots-ai-agents-will-come-to-earth-in-2025/.
[114] Robison, K., ‘Agents are the Future AI Companies Promise — and Desperately Need’, The Verge, 2024, available at https://www.theverge.com/2024/10/10/24266333/ai-agents-assistants-openai-google-deepmind-bots.
[115] Hess, K., Fedasiuk, R. and Busby, J., ‘Through the Chat Window and Into the Real World: Preparing for AI Agents’, Center for Security and Emerging Technology, 2024, available at https://cset.georgetown.edu/publication/through-the-chat-window-and-into-the-real-world-preparing-for-ai-agents/.
[116] METR, ‘Measuring AI Ability to Complete Long Tasks’, Measuring Evaluation and Testing Research, 2025, available at https://metr.org/blog/2025-03-19-measuring-ai-ability-to-complete-long-tasks/.
[117] Hess, K., Fedasiuk, R. and Busby, J., ‘Through the Chat Window and Into the Real World: Preparing for AI Agents’, Center for Security and Emerging Technology, 2024, available at https://cset.georgetown.edu/publication/through-the-chat-window-and-into-the-real-world-preparing-for-ai-agents/.
[118] AI Digest, ‘Beyond Chat: AI Agent Demo’, AI Digest, available at http://bit.ly/41tcyEM.
[119] Exponential View, ‘From ChatGPT to a Billion Agents’, Exponential View, 2024, available at https://www.exponentialview.co/p/from-chatgpt-to-a-billion-agents.
[120] Artificial Analysis, ‘Comparison of AI Models Across Intelligence, Performance, Price’, Artificial Analysis, available at https://substack.com/redirect/2d183110-b7a7-4455-88ae-183f160ae126?j=eyJ1IjoiNjRwZWIifQ.rPJDWzsJS7pjXktDI00JgjUPHcgYbL9gv7-14JlO46k.
[121] Goldman, S., ‘A Year Ago, DeepMind’s AlphaFold AI Changed the Shape of Science — but There Is More Work to Do’, VentureBeat, 2023, available at https://substack.com/redirect/dda4106f-2aed-4b73-8163-12c94a75a024?j=eyJ1IjoiNjRwZWIifQ.rPJDWzsJS7pjXktDI00JgjUPHcgYbL9gv7-14JlO46k.
[122] Hynda, Z., ‘The History of American Agriculture’, Ohio State University, 2022, available at https://u.osu.edu/beef/2022/07/06/the-history-of-american-agriculture/.
[123] Hoppe, R., ‘Increases in Labor Quality Contributed to Growth in U.S. Agricultural Output’, U.S. Department of Agriculture, Economic Research Service, 2022, available at https://www.ers.usda.gov/amber-waves/2022/february/increases-in-labor-quality-contributed-to-growth-in-u-s-agricultural-output.
[124] Macnamara, B. et al., ‘Does Using Artificial Intelligence Assistance Accelerate Skill Decay and Hinder Skill Development Without Performers’ Awareness?’, Cogn Res Princ Implic, vol. 9, no. 1, 2024, p. 46, available at https://pmc.ncbi.nlm.nih.gov/articles/PMC11239631/.
[125] Aviation Rulemaking Committee, ‘ARC Recommendation 16-9: Guidance Material for Part 23 Accepted Means of Compliance Based on ASTM Consensus Standards’, Federal Aviation Administration, 2016, available at https://www.faa.gov/sites/faa.gov/files/about/office_org/headquarters_offices/avs/ACT_ARC_Reco_16-9.pdf.
[126] Okaneme, E.C. and Okaneme, G., ‘Cognitive Biases in the Development of Artificial Intelligence’, Societies, vol. 15, no. 1, 2024, p. 6, available at https://doi.org/10.3390/soc15010006.
[127] Barron’s, ‘Nvidia CEO Jensen Huang Says 2025 Is the Year of AI Agents’, Barron’s, 2025, available at https://www.barrons.com/articles/nvidia-stock-ceo-ai-agents-8c20ddfb.
[128] Hess, K., Fedasiuk, R. and Busby, J., ‘Through the Chat Window and Into the Real World: Preparing for AI Agents’, Center for Security and Emerging Technology, 2024, available at https://cset.georgetown.edu/publication/through-the-chat-window-and-into-the-real-world-preparing-for-ai-agents/.
[129] Wang, G. et al., ‘Voyager: An Open-ended Embodied Agent with Large Language Models’, ArXiv, 2023, available at https://arxiv.org/pdf/2305.16291.
[130] OpenAI, ‘Introducing Operator’, OpenAI, 2025, available at https://openai.com/index/introducing-operator/.
[131] Business Insider, ‘What to Know About Anthropic’s AI Tool That Can Control Your Computer’, Business Insider, 2024, available at https://www.businessinsider.com/anthropic-claude-computer-use-ai-explainer-2024-10.
[132] Google, ‘Google Introduces Gemini 2.0: A New AI Model for the Agentic Era’, Google, 2024, available at https://blog.google/technology/google-deepmind/google-gemini-ai-update-december-2024/.
[133] Lin, S., Hilton, J., and Evans, O., ‘TruthfulQA: Measuring How Models Mimic Human Falsehoods’, arXiv, 2021, https://arxiv.org/abs/2109.07958
[134] OpenReview, ‘PlanBench: An Extensible Benchmark for Evaluating Large Language Models on Planning and Reasoning about Change’, OpenReview, 2023, available at https://openreview.net/forum?id=YXogl4uQUO.
[135] Valmeekam, K., Stechly, K. and Kambhampati, S., ‘LLMs Still Can’t Plan; Can LRMs? A Preliminary Evaluation of OpenAI’s o1 on PlanBench’, ArXiv, 2024, available at https://arxiv.org/abs/2409.13373.
[136] Google DeepMind, ‘Project Mariner’, Google DeepMind, 2024, available at http://deepmind.google/technologies/project-mariner.
[137] He, H. et al., ‘WebVoyager: Building an End-to-End Web Agent with Large Multimodal Models’, ArXiv, 2024, available at https://arxiv.org/abs/2401.13919.
[138] Nouwou Mindom, P.S., Nikanjam, A. and Khomh, F., ‘Harnessing Pre-trained Generalist Agents for Software Engineering Tasks’, arXiv, 2023, available at https://arxiv.org/abs/2312.15536.
[139] Garcia, X., et al., ‘The unreasonable effectiveness of few-shot learning for machine translation’, arXiv, 2023, available at https://arxiv.org/abs/2302.01398
[140] Kanjee, Z., Crowe, B. and Rodman, A., ‘Accuracy of a Generative Artificial Intelligence Model in a Complex Diagnostic Challenge’, JAMA, 2023, available at https://jamanetwork.com/journals/jama/fullarticle/2806457.
[141] Google, ‘Introducing Pathways: A Next‑generation AI Architecture’, Google, 2021, https://blog.google/technology/ai/introducing-pathways-next-generation-ai-architecture/#:~:text=Today%27s%20AI%20models%20are%20typically,thousands%20or%20millions%20of%20things
[142] Romero, A., ‘GPT-4: The Bitterer Lesson’, The Algorithmic Bridge, 2023, https://www.thealgorithmicbridge.com/p/gpt-4-the-bitterer-lesson
[143] Lin, S., Hilton, J., and Evans, O., ‘TruthfulQA: Measuring How Models Mimic Human Falsehoods’, arXiv, 2021, https://arxiv.org/abs/2109.07958
[144] Somers, J., ‘A Revolution in How Robots Learn’, The New Yorker, 2024, https://www.newyorker.com/magazine/2024/12/02/a-revolution-in-how-robots-learn
[145] ‘ARC Prize – What Is ARC-AGI?’, ARC Prize, n.d., https://arcprize.org/arc
[146] ‘OpenAI o3 Breakthrough High Score On ARC-AGI-pub’, ARC Prize, 2024, https://arcprize.org/blog/oai-o3-pub-breakthrough
[147] LeGris, S., Vong, W. K., Lake, B. M., and Gureckis, T. M., ‘H-ARC: A Robust Estimate of Human Performance on the Abstraction and Reasoning Corpus Benchmark’, arXiv, 2024, https://arxiv.org/pdf/2409.01374
[148] Galatzer-Levy, I. R., et al., ‘The Cognitive Capabilities Of Generative AI: A Comparative Analysis With Human Benchmarks’, arXiv, 2024, https://arxiv.org/abs/2410.07391
[149] Goodside, R., ‘ARC-AGI scores for past five years…’, X, 2024, https://x.com/goodside/status/1870243391814152544
[150] ‘IDAIS-Beijing – International Dialogues On AI Safety’, IDAIS, 2024, https://idais.ai/dialogue/idais-beijing/
[151] Kalliamvakou, E., ‘Research: Quantifying GitHub Copilot’s Impact on Developer Productivity and Happiness’, GitHub Blog, 2024, https://github.blog/news-insights/research/research-quantifying-github-copilots-impact-on-developer-productivity-and-happiness/
[152] Owen, D., ‘Interviewing AI Researchers On Automation Of AI R&D’, Epoch AI, 2024, https://epoch.ai/blog/interviewing-ai-researchers-on-automation-of-ai-rnd
[153] Krishnan, N., ‘OpenAI’s O3: A New Frontier in AI Reasoning Models’, Towards AI, 2024, https://pub.towardsai.net/openais-o3-a-new-frontier-in-ai-reasoning-models-a02999246ffe
[154] Wijk, H. et al., ‘Re-bench: Evaluating Frontier AI R&D Capabilities Of Language Model Agents Against Human Experts’, ArXiv, 2024, https://arxiv.org/abs/2411.15114
[155] Lin, T., ‘Measuring Automated Kernel Engineering’, METR, 2025, https://metr.org/blog/2025-02-14-measuring-automated-kernel-engineering/
[156] Afifi-Sabet, K., ‘New AGI Benchmark Indicates Whether A Future AI Model Could Cause ‘catastrophic Harm”, Live Science, 2024, https://www.livescience.com/technology/artificial-intelligence/scientists-design-new-agi-benchmark-that-may-say-whether-any-future-ai-model-could-cause-catastrophic-harm
[157] Huang, Q., Vora, J., Liang, P., and Leskovec, J., ‘MLAgentBench: Evaluating Language Agents on Machine Learning Experimentation’, arXiv, 2024, https://arxiv.org/pdf/2310.03302
[158] Owen, D., ‘Interviewing AI Researchers On Automation Of AI R&D’, Epoch AI, 2024, https://epoch.ai/blog/interviewing-ai-researchers-on-automation-of-ai-rnd
[159] Berg, J.E., Nelson, F.D. and Rietz, T.A., ‘Prediction Market Accuracy in the Long Run’, International Journal of Forecasting, vol. 24, 2008, available at: https://www.sciencedirect.com/science/article/abs/pii/S0169207008000320.
[160] Cowgill, B. and Zitzewitz, E., ‘Corporate Prediction Markets: Evidence from Google, Ford, and Firm X’, The Review of Economic Studies, vol. 82, 2015, available at: https://www.jstor.org/stable/43869468.
[161] Tetlock, P.E., Mellers, B.A., Rohrbaugh, N. and Chen, E., Forecasting tournaments: Tools for increasing transparency and improving the quality of debate, 2014, Current Directions in Psychological Science, 23(4), pp.290-295. https://journals.sagepub.com/doi/abs/10.1177/0963721414534257
[162] Manifold, ‘Will AI be Recursively Self Improving by mid 2026?’, Manifold, n.d., https://manifold.markets/MaxHarms/will-ai-be-recursively-self-improvi
[163] Manifold, ‘Will models be able to do the work of an AI researcher/engineer before 2027?’, Manifold, n.d., https://manifold.markets/Tossup/lasa-will-models-be-able-to-do-the
[164] Metaculus, ‘When will AIs program programs that can program AIs?’, Metaculus, n.d., https://www.metaculus.com/questions/406/date-ais-capable-of-developing-ai-software/
[165] Trammell, P. and Korinek, A., ‘Economic Growth under Transformative AI’, NBER Working Paper No. 31815, National Bureau of Economic Research, October 2023, https://www.nber.org/papers/w31815
[166] Trammell, P. and Korinek, A., ‘Economic Growth under Transformative AI’, NBER Working Paper No. 31815, National Bureau of Economic Research, October 2023, https://www.nber.org/papers/w31815
[167] Korinek, A., ‘Economic Policy Challenges for the Age of AI’, National Bureau of Economic Research, 2024, https://www.nber.org/system/files/working_papers/w32980/w32980.pdf
[168] UK Government, ‘International Scientific Report on the Safety of Advanced AI Interim Report’, UK Government, 2024, https://assets.publishing.service.gov.uk/media/6716673b96def6d27a4c9b24/international_scientific_report_on_the_safety_of_advanced_ai_interim_report.pdf
[169] UK Government, ‘International AI Safety Report’, UK Government, 2025, https://assets.publishing.service.gov.uk/media/679a0c48a77d250007d313ee/International_AI_Safety_Report_2025_accessible_f.pdf
[170] UK Government, ‘International AI Safety Report’, UK Government, 2025, https://assets.publishing.service.gov.uk/media/679a0c48a77d250007d313ee/International_AI_Safety_Report_2025_accessible_f.pdf
[171] UK Government, ‘International AI Safety Report’, UK Government, 2025, https://assets.publishing.service.gov.uk/media/679a0c48a77d250007d313ee/International_AI_Safety_Report_2025_accessible_f.pdf
[172] Kaplan, J. et al., ‘Scaling Laws for Neural Language Models’, arXiv, 2020. Available at: https://arxiv.org/abs/2001.08361
[173] Sevilla, J. et al., ‘Can AI Scaling Continue Through 2030?’, Epoch AI, 2024. Available at: https://epoch.ai/blog/can-ai-scaling-continue-through-2030
[174] Sevilla, J. et al., ‘Can AI Scaling Continue Through 2030?’, Epoch AI, 2024. Available at: https://epoch.ai/blog/can-ai-scaling-continue-through-2030
[175] Sevilla, J. et al., ‘Can AI Scaling Continue Through 2030?’, Epoch AI, 2024. Available at: https://epoch.ai/blog/can-ai-scaling-continue-through-2030
[176] Sevilla, J. et al., ‘Can AI Scaling Continue Through 2030?’, Epoch AI, 2024. Available at: https://epoch.ai/blog/can-ai-scaling-continue-through-2030
[177] Sevilla, J. et al., ‘Can AI Scaling Continue Through 2030?’, Epoch AI, 2024. Available at: https://epoch.ai/blog/can-ai-scaling-continue-through-2030
[178] Juijn, D., ‘No, Deep Learning Is (probably) Not Hitting A Wall’, Centre for Future Generations, 2024. Available at: https://cfg.eu/no-deep-learning-is-probably-not-hitting-a-wall/
[179] Hu, K. and Tong, A., ‘OpenAI and others seek new path to smarter AI as current methods hit limitations’, Reuters, 2024. Available at: https://www.reuters.com/technology/artificial-intelligence/openai-rivals-seek-new-path-smarter-ai-current-methods-hit-limitations-2024-11-11/
[180] Metz, R. et al., ‘OpenAI, Google and Anthropic Are Struggling to Build More Advanced AI’, Bloomberg, 2024. Available at: https://www.bloomberg.com/news/articles/2024-11-13/openai-google-and-anthropic-are-struggling-to-build-more-advanced-ai
[181] Marcus, G., ‘Folks, Game Over….’, X, 2024. Available at: https://x.com/GaryMarcus/status/1855382564015689959
[182] Patel, D., ‘John Schulman (OpenAI Cofounder) – Reasoning, RLHF, & Plan For 2027 AGI’, YouTube, 2024. Available at: https://www.youtube.com/watch?v=Wo95ob_s_NI
[183] OpenAI, ‘Introducing OpenAI o1-preview’, OpenAI, 2024. Available at: https://openai.com/index/introducing-openai-o1-preview/
[184] Epoch AI, ‘FrontierMath: LLM Benchmark For Advanced AI Math Reasoning’, Epoch AI, 2024. Available at: https://epoch.ai/frontiermath
[185] Epoch AI, ‘FrontierMath: LLM Benchmark For Advanced AI Math Reasoning’, Epoch AI, 2024. Available at: https://epoch.ai/frontiermath
[186] Priestley, M., Sluckin, T.J. and Tiropanis, T., ‘Innovation on the Web: the end of the S-curve?’, Internet Histories, vol. 4, 2020. Available at: https://www.researchgate.net/publication/340525808_Innovation_on_the_Web_the_end_of_the_S-curve
[187] Vectara, ‘Hallucination Leaderboard’, GitHub, n.d., https://github.com/vectara/hallucination-leaderboard
[188] Gurnani, R. et al., ‘AI-assisted discovery of high-temperature dielectrics for energy storage’, Nature, 2024, https://www.nature.com/articles/s41467-024-50413-x.pdf
[189] Lin, S., Hilton, J., and Evans, O., ‘TruthfulQA: Measuring How Models Mimic Human Falsehoods’, arXiv, 2021, https://arxiv.org/abs/2109.07958
[190] Evans, O., Chua, J., and Lin, S., ‘New, Improved Multiple‑choice TruthfulQA’, Lesswrong, 2025, https://www.lesswrong.com/posts/Bunfwz6JsNd44kgLT/new-improved-multiple-choice-truthfulqa
[191] Armstrong, S. and Sotala, K., ‘How We’re Predicting AI—or Failing To’, Machine Intelligence Research Institute, 2012, https://intelligence.org/files/PredictingAI.pdf
[192] Pillay, T., ‘How OpenAI’s Sam Altman Is Thinking About AGI and Superintelligence in 2025’, TIME, 2025, https://time.com/7205596/sam-altman-superintelligence-agi/.
[193] Fridman, L., ‘Dario Amodei: Anthropic CEO On Claude, AGI & The Future Of AI & Humanity | Lex Fridman Podcast #452’, YouTube, 2024, https://www.youtube.com/watch?v=ugvHCXCOmm4.
[194] CNBC Television, ‘Anthropic CEO: More confident than ever that we’re ‘very close’ to powerful AI capabilities’, YouTube, 2025, https://www.youtube.com/watch?v=7LNyUbii0zw&t=134s.
[195] Yee, L., Chui, M., and Roberts, R., with Xu, S., ‘Why agents are the next frontier of generative AI’, McKinsey Digital, 2024, https://www.mckinsey.com/capabilities/mckinsey-digital/our-insights/why-agents-are-the-next-frontier-of-generative-ai.
[196] Chui, M. et al., ‘The economic potential of generative AI’, McKinsey, 2023, https://www.mckinsey.com/~/media/mckinsey/business%20functions/mckinsey%20digital/our%20insights/the%20economic%20potential%20of%20generative%20ai%20the%20next%20productivity%20frontier/the-economic-potential-of-generative-ai-the-next-productivity-frontier.pdf.
[197] Chui, M. et al., ‘The economic potential of generative AI’, McKinsey, 2023, https://www.mckinsey.com/~/media/mckinsey/business%20functions/mckinsey%20digital/our%20insights/the%20economic%20potential%20of%20generative%20ai%20the%20next%20productivity%20frontier/the-economic-potential-of-generative-ai-the-next-productivity-frontier.pdf.
[198] Chui, M. et al., ‘The economic potential of generative AI’, McKinsey, 2023, https://www.mckinsey.com/~/media/mckinsey/business%20functions/mckinsey%20digital/our%20insights/the%20economic%20potential%20of%20generative%20ai%20the%20next%20productivity%20frontier/the-economic-potential-of-generative-ai-the-next-productivity-frontier.pdf.
[199] Grace, K. et al., ‘Thousands of AI Authors on the Future of AI’, arXiv, 2024, https://arxiv.org/abs/2401.02843.
[200] Tetlock, P.E., Mellers, B.A., Rohrbaugh, N. and Chen, E., Forecasting tournaments: Tools for increasing transparency and improving the quality of debate, 2014, Current Directions in Psychological Science, 23(4), pp.290-295. https://journals.sagepub.com/doi/abs/10.1177/0963721414534257
[201] Berg, J.E., Nelson, F.D. and Rietz, T.A., ‘Prediction Market Accuracy in the Long Run’, International Journal of Forecasting, vol. 24, 2008, available at: https://www.sciencedirect.com/science/article/abs/pii/S0169207008000320.
[202] Cowgill, B. and Zitzewitz, E., ‘Corporate Prediction Markets: Evidence from Google, Ford, and Firm X’, The Review of Economic Studies, vol. 82, 2015, available at: https://www.jstor.org/stable/43869468.
[203] AI Digest, ‘Timeline of AI Forecasts’, AI Digest, 2025, available at: https://theaidigest.org/timeline.
[204] Metaculus, ‘When Will the First Weakly General AI System Be Devised, Tested, and Publicly Announced?’, Metaculus, n.d., available at: https://www.metaculus.com/questions/3479/date-weakly-general-ai-is-publicly-known/.
[205] Metaculus, ‘When Will the First Weakly General AI System Be Devised, Tested, and Publicly Announced?’, Metaculus, n.d., available at: https://www.metaculus.com/questions/3479/date-weakly-general-ai-is-publicly-known/.
[206] Metaculus, ‘Will an AI Be Able to Reliably Construct Bug‑free Code of More Than 10,000 Lines Before 2030?’, Metaculus, n.d., available at: https://www.metaculus.com/questions/11188/ai-programming-competency-before-2030/.
[207] Janků, D., Reddel, M., Yampolskiy, R. and Hausenloy, J., ‘We Have No Science of Safe AI’, Centre for Future Generations, 2024, available at: https://cfg.eu/we-have-no-science-of-safe-ai/.