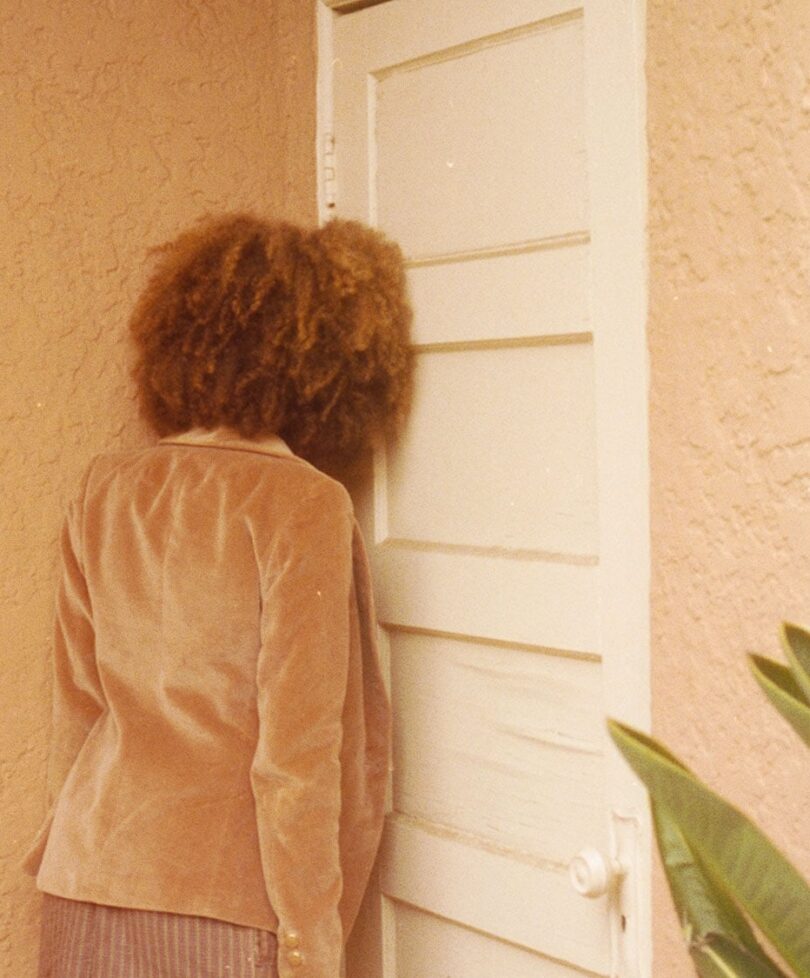
No, deep learning is (probably) not hitting a wall
Daan Juijn argues that contrary to current news reports, AI is not hitting a wall. Instead, the AI development process is shifting – with important implications for AI governance.
Ilya Sutskever, a co-founder of OpenAI, told Reuters that the advances from scaling up pre-training have plateaued. Leaks to reputable media outlets indicate that this trend is occurring across the industry, including at leading companies like OpenAI, Google DeepMind, and Anthropic. This has prompted prominent sceptics of the current paradigm that “deep learning is hitting a wall.”
Is this claim true? And what implications would these developments hold for the governance of advanced AI? As is often the case, reality is more nuanced than headlines suggest, and it would be premature to be sceptical of rapid advancements in AI capabilities. Pre-training is only one part of the AI development process, and we are seeing continuing gains in other areas like post-training. Furthermore, with the release of OpenAI’s o1 model family, the industry has entered a new paradigm. New types of general reasoning models can now achieve better performance by ‘thinking for longer’ after the training process is completed.
This new era of scaling so-called ‘runtime compute’ has the potential to reshape the AI landscape, introducing greater uncertainty to its trajectory. Innovations emerging from this volatile period could profoundly influence the field’s direction for years to come. The unfolding paradigm shift poses challenges to future-proof policymaking but also creates unique opportunities. Policymakers should seize this transitional moment to prioritise investments in fundamental research that enhance the trustworthiness of AI systems. For example, emerging techniques could allow us to analyse the “chains of thought” produced by these advanced reasoning models during runtime, offering a window into their inner workings and enabling the detection of previously hidden misbehaviour.
There’s more to AI than pre-training
What does it mean if the ‘returns from scaling up pre-training have plateaued’? It is tempting to interpret Sutskever’s claim as a sign that AI progress has hit a hard ceiling. However, this would be a mistake. To fully understand the implications of his recent news reports, let’s revisit the broader AI training process.
Modern AI systems have evolved far past simple next-word predictors. Instead, they are sophisticated software products built on top of foundational models, with training pipelines that extend significantly beyond pre-training. One critical step in this evolution is the addition of post-training (which involves multiple techniques such as reinforcement learning from human feedback). These techniques are used to turn pre-trained models with rich learned representations into helpful, harmless and honest assistants.
Post-training methods are also increasingly addressing other significant shortcomings in pre-training. For example, pre-training datasets often lack high-quality examples of reasoning and planning, which leaves models prone to errors like hallucinations and limits their usefulness as agents or in formal domains like mathematics. Additional reinforcement learning techniques are now being applied during post-training to fill these gaps, resulting in rapid progress in areas like software engineering. Anthropic CEO Dario Amodei recently predicted that post-training could soon overtake pre-training in terms of compute costs—a testament to its growing importance.
A New Paradigm
Perhaps even more transformative is the new paradigm introduced by OpenAI’s o1 model family. This innovation allows models to ‘think for longer’ after the training process is completed. By leveraging more compute at runtime, models can refine their reasoning, spot mistakes and pursue different paths, thereby generating more accurate responses. Importantly, this approach also creates a feedback loop for post-training. By thinking for longer at runtime, models are more likely to achieve correct answers, which in turn streamlines the reinforcement learning process and helps verify the accuracy of other AI-generated outputs. Automatic verification may in turn enable a more general type of self-play, which has previously been applied to achieve superhuman performance in narrow applications like the game of Go.
In summary, Sutskever’s claim about plateauing pre-training gains highlights a shift in the development pipeline. Pre-training represents only one part of the process. Recent rapid advances in post-training and runtime techniques suggest that overall progress is far from over. It also implies that compute may remain as central to AI developments as it has been for the past decade. As Sutskever himself stated: “Scaling the right thing matters more now than ever.”
To illustrate the nuance here, consider this analogy: the current state of affairs in AI is similar to that in the car industry some fifteen years ago. The environmental gains from improving combustion engines have slowed, but at the same time, the first hybrids and EVs are entering the market.
An age of wonders and discovery
All this isn’t to say that the plateauing of pre-training gains should be dismissed as irrelevant. On the contrary, this shift has significant implications for the trajectory of advanced AI, the development landscape and AI governance. For years, scaling up pre-training compute has been the dominant strategy for advancing capabilities. If these gains are now indeed diminishing, the industry will need to explore new methods to continue translating compute increases into meaningful improvements. This transition introduces a higher degree of uncertainty about the future rate of progress.
It may also lead to larger disparities between AI companies. After all, not all frontier AI companies seem to have solved the challenges associated with this new emerging paradigm. While OpenAI’s o1 model demonstrates a promising approach, algorithmic secrets are closely guarded. This could lead to uneven progress across the industry, with some labs pulling ahead. It could also result in companies developing distinct solutions to the hurdles they face, possibly leading to diverging products, new niches and a wider range of policy challenges.
AI governance, then, has to become more adaptive and forward-looking. How can regulations effectively address development pipelines that are becoming increasingly specific to individual labs? Are our current evaluation methods—designed to detect potentially dangerous capabilities—adequate for the challenges ahead? And how should regulators make use of training compute thresholds in the context of emerging runtime compute techniques? The latter issue is especially urgent given the EU AI Act’s reliance on training compute thresholds for general-purpose models with systemic risks. Are these one-dimensional thresholds still sufficient, or should they be broadened to include runtime compute metrics as well?
This moment of transition also presents unique opportunities. For instance, runtime techniques often rely on letting a model output extensive ‘chains of thought’. If we can increase the faithfulness of such internal model monologues, this may present a way to ‘peek inside the model’ and test for deception or biases.
With AI at the brink of a new paradigm, now is the time to prioritise fundamental research aimed at making AI systems more trustworthy, reliable, and aligned with human values. After all, innovations made during this volatile period could shape the direction of the field for years to come. In the words of Sutskever: “The 2010s were the age of scaling, now we’re back in the age of wonder and discovery once again.”